High Performance Computing Publications
Papers, research, and articles made possible by the HPC team and HPC resources
Publications by Patricia Kovatch
Dean for Scientific Computing and Data Patricia Kovatch contributes to scientific journals and publications as a collaborative and primary author. Read more publications.
Excerpt: Development of a federated learning approach to predict acute kidney injury in adult hospitalized patients with COVID-19 in New York City
Federated learning is a technique for training predictive models without sharing patient-level data, thus maintaining data security while allowing inter-institutional collaboration. We used federated learning to predict acute kidney injury within three and seven days of admission, using demographics, comorbidities, vital signs, and laboratory values, in 4029 adults hospitalized with COVID-19 at five sociodemographically diverse New York City hospitals… Read more
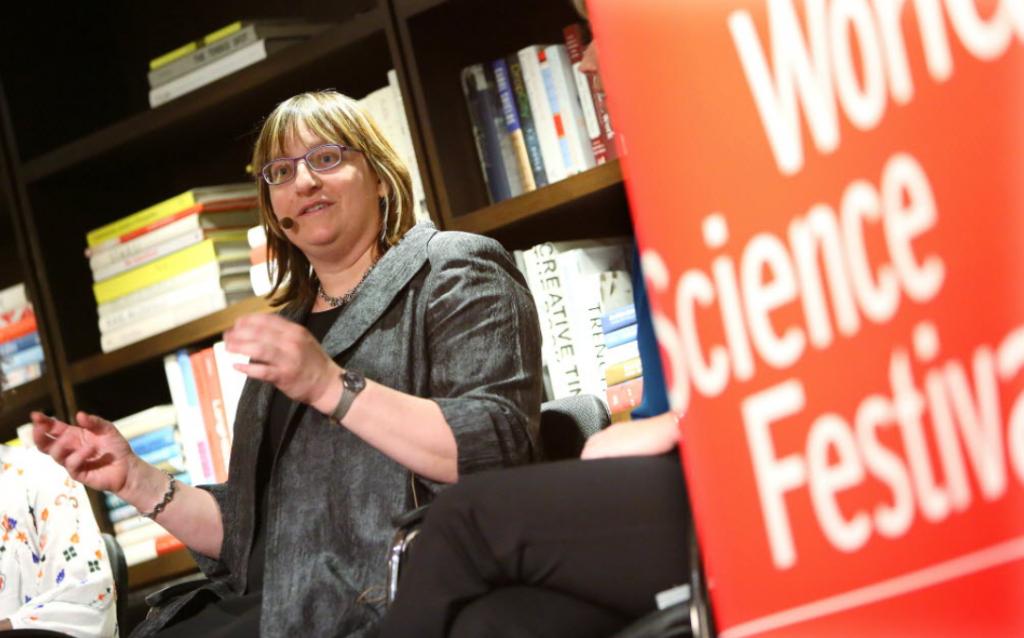
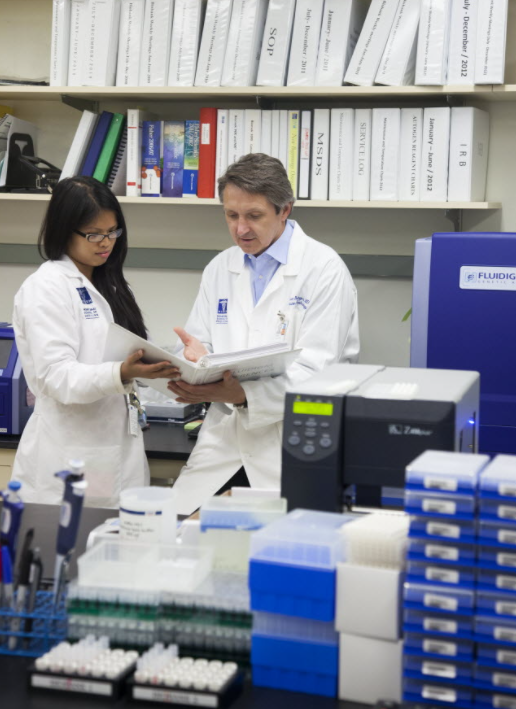
Publications Through Minerva
Minerva’s computing power helps scientists and researchers advance their studies. Many publications feature work made possible through Minerva.
Abstract: Deep learning identified pathological abnormalities predictive of graft loss in kidney transplant biopsies
by Yi Z, Salem F, Menon MC, Keung K, Xi C, et al. February 2022 | Interstitial fibrosis, tubular atrophy, and inflammation are major contributors to kidney allograft failure. Here we sought an objective, quantitative pathological assessment of these lesions to improve predictive utility and constructed a deep-learning-based pipeline recognizing normal vs. abnormal kidney tissue compartments and mononuclear leukocyte infiltrates. Periodic acid- Schiff stained slides of transplant biopsies (60 training and 33 testing) were used to quantify pathological lesions specific for interstitium, tubules and mononuclear leukocyte infiltration. DOI: 10.1016/j.kint.2021.09.028
Research at Mount Sinai
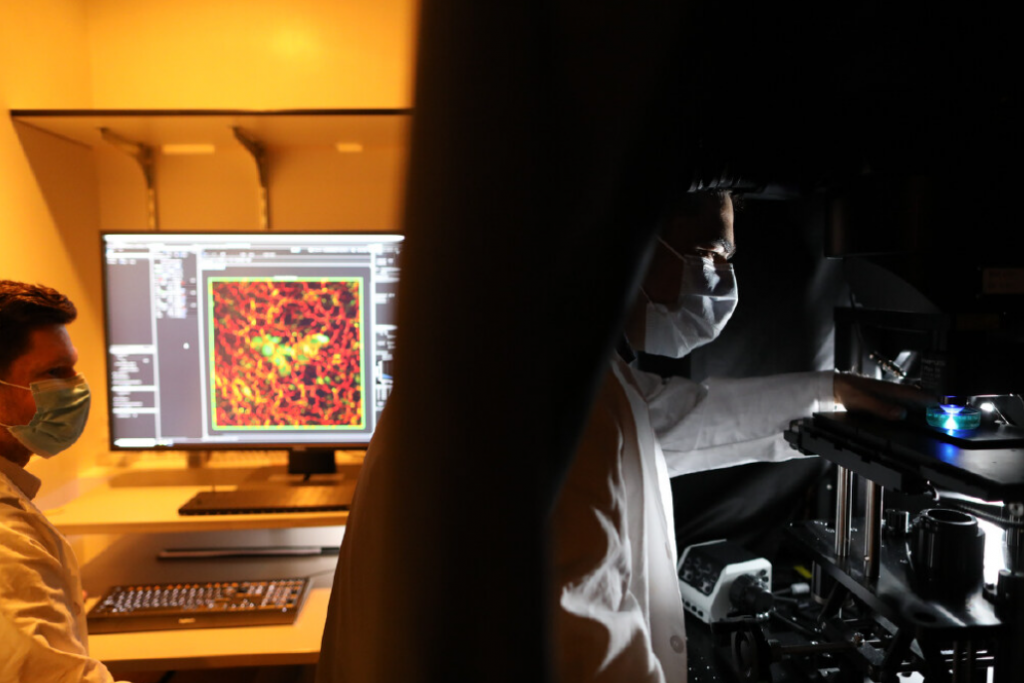
Acknowledge Mount Sinai in Your Work
Utilizing S10 BODE and CATS partitions requires acknowledgements of support by NIH in your publications. To assist, we have provided exact wording of acknowledgements required by NIH for your use.
Click here for acknowledgements.
Supported by grant UL1TR004419 from the National Center for Advancing Translational Sciences, National Institutes of Health.