What’s Our Vision?
Give us your genome, and we will accurately predict your disease risks and drug targets—so you can benefit from personalized diagnostics, prevention, and treatment.
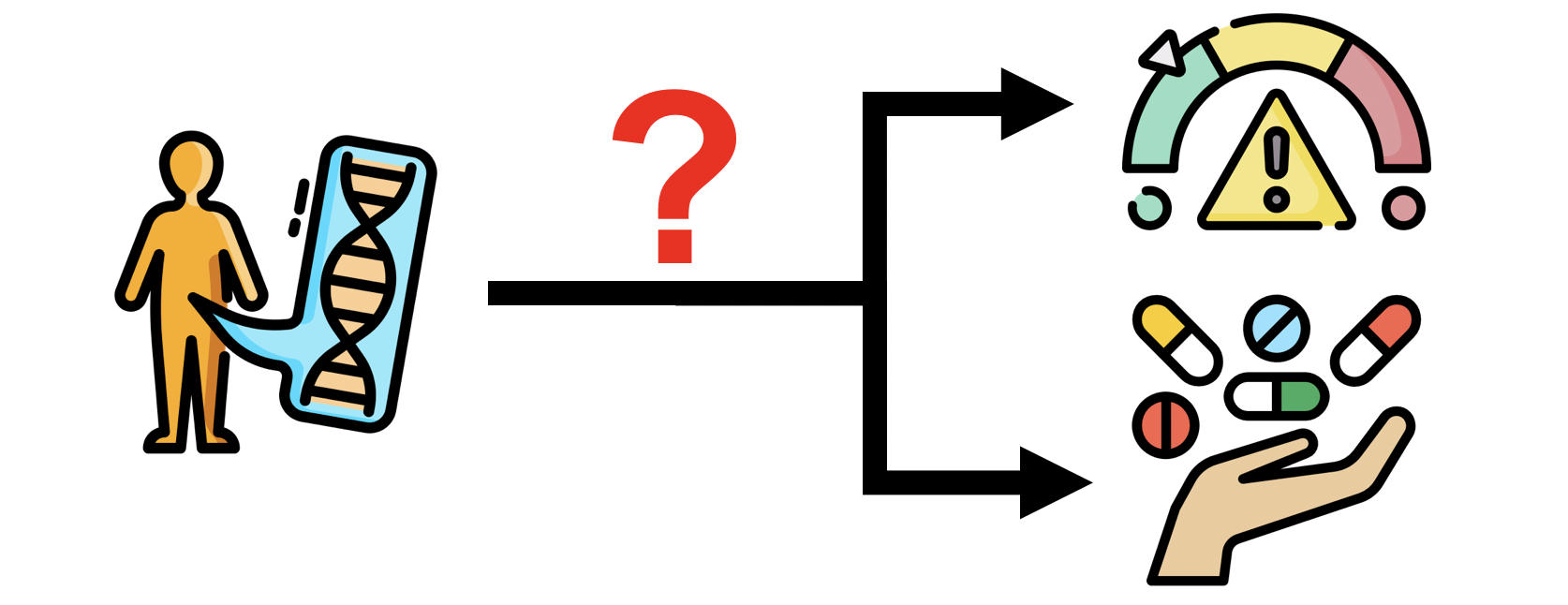
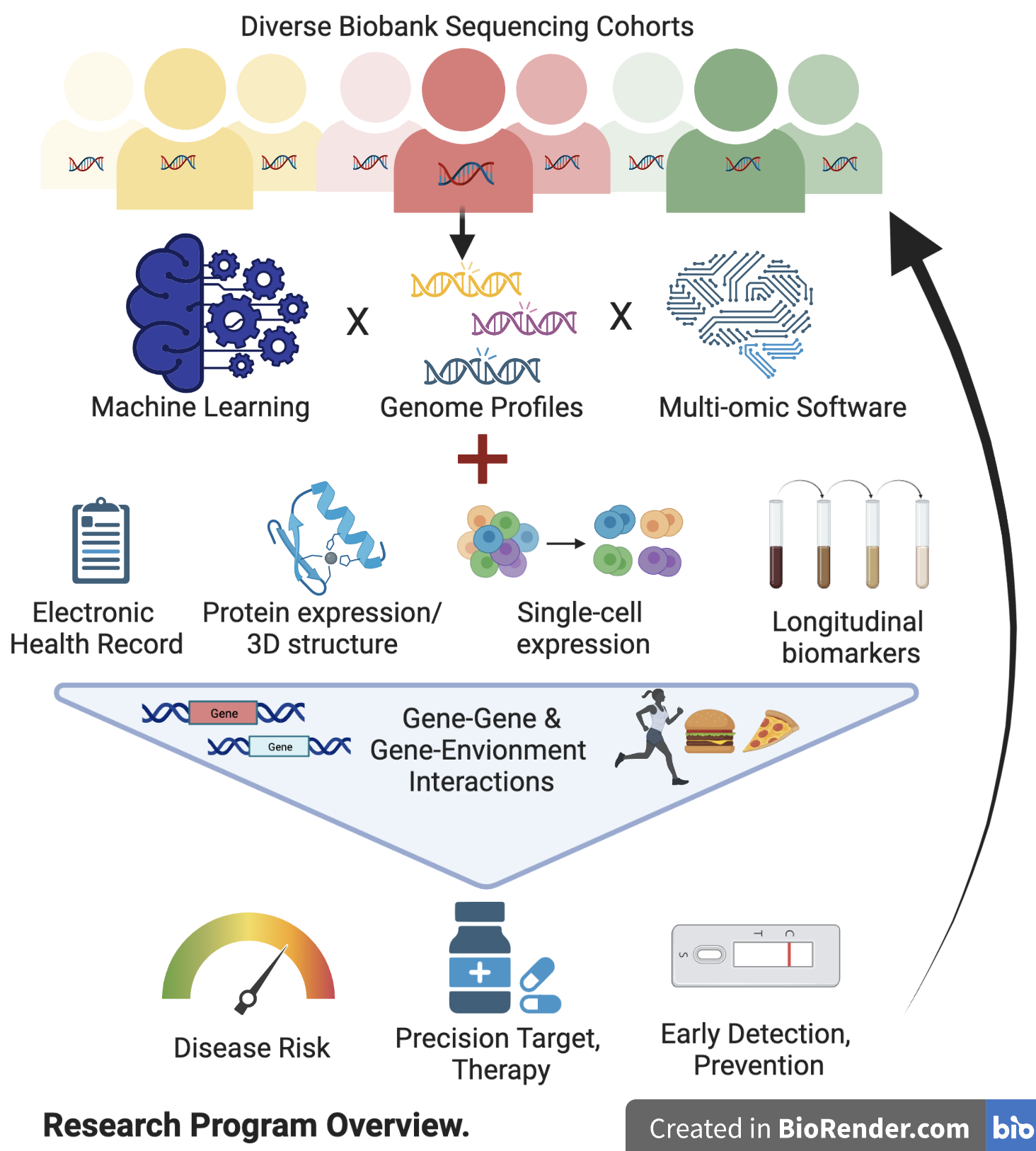
How do we get there?
Here are selected examples of the scientific research done by our team:
We identified common risk variants in Alzheimer’s disease (Nat Neuro 2017) and rare pathogenic variants in Cancer (Cell 2018) while leading national/international genomics consortia projects.
We specifically characterized genomes of patients from diverse ancestry populations (Cancer Cell 2020, Genome Med 2020, Genome Med 2021): to ensure that we’re advancing precision medicine for all.
We linked the identified mutations to likely treatment responses to targeted or immuno-therapy (Cell Rep Med 2021, Cancers 2021, Cell Reports 2021).
To further bridge the gaps between genetics and treatments, we integrated proteomics data to validate genomic findings and identify new drug targets (Nat Comm 2017, Front Onc 2022).
To decode downstream impacts of genetic variants, we built multi-omics software (MCP 2019, Nat Comm 2021, PSB 2021, Comm Bio 2021) and publicly shared all codes.
PI Huang’s first/co-first author research article: (bold); last-author research article: (bold italic)
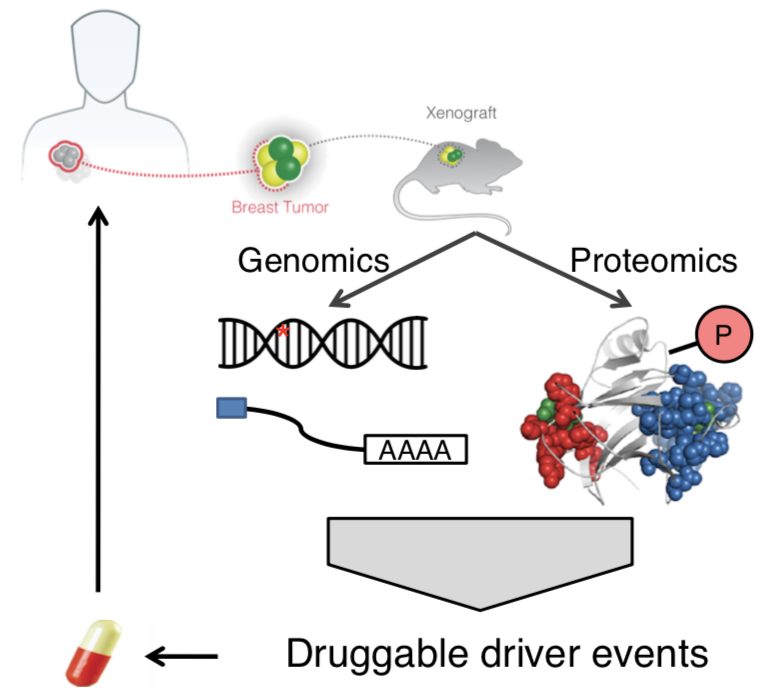
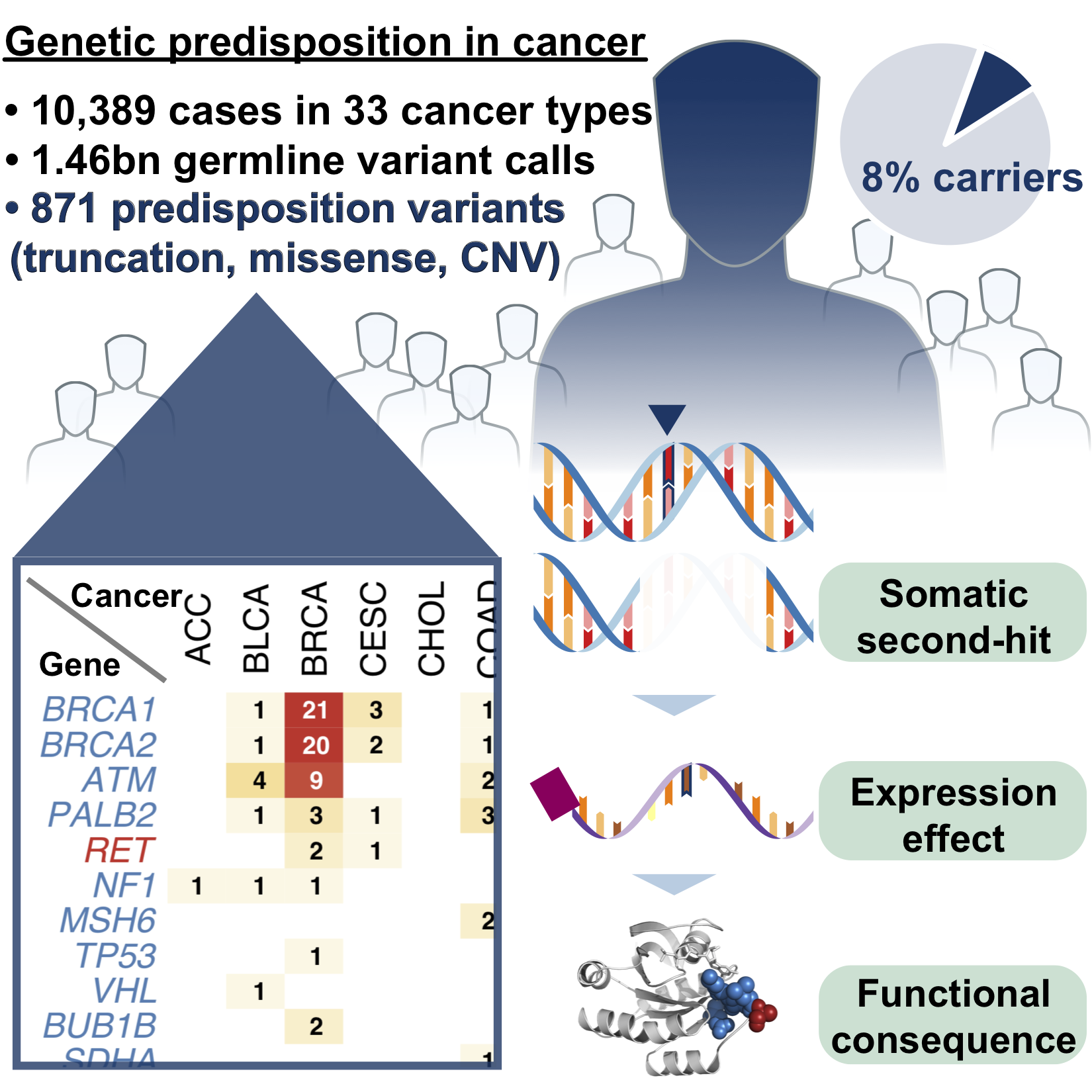
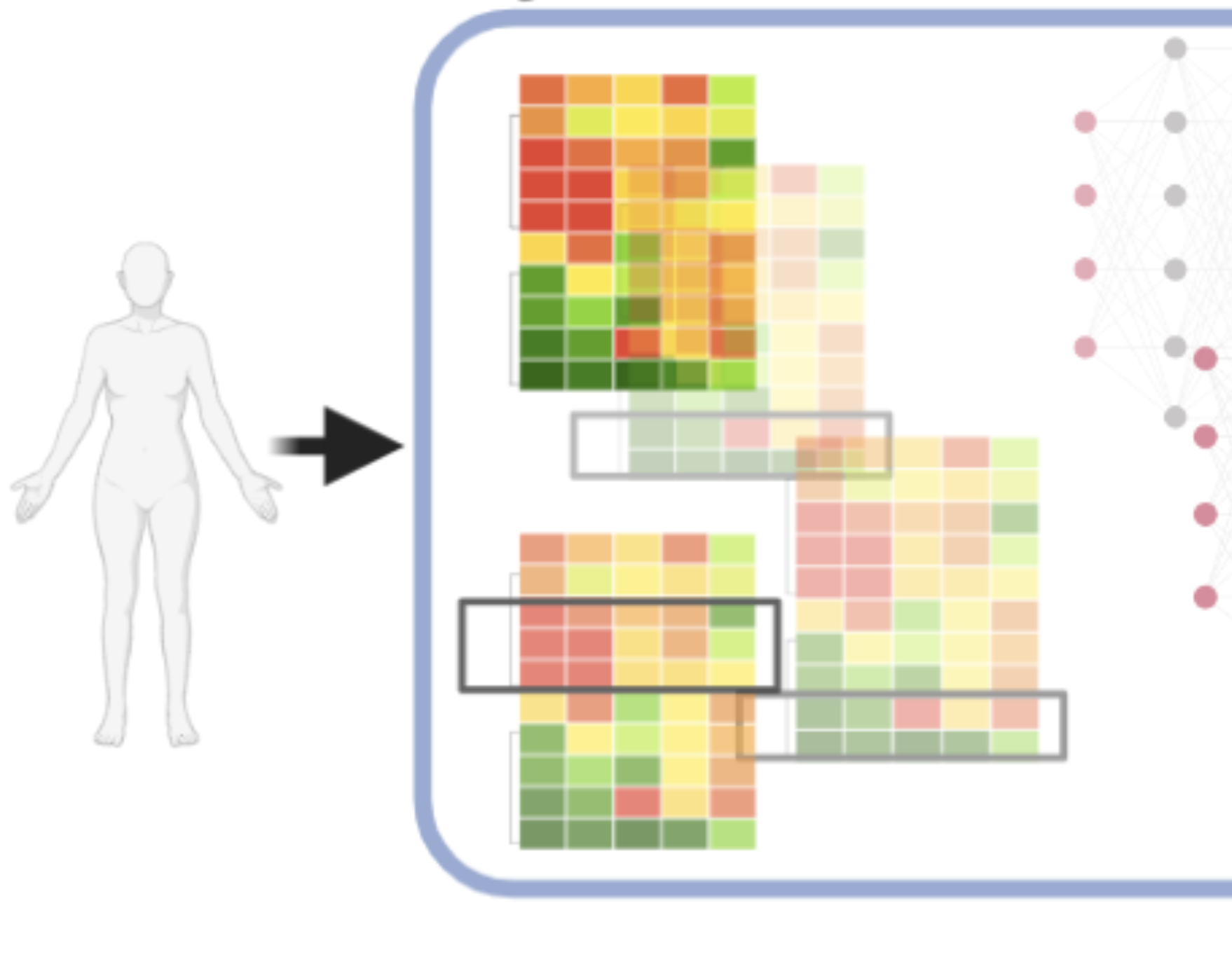
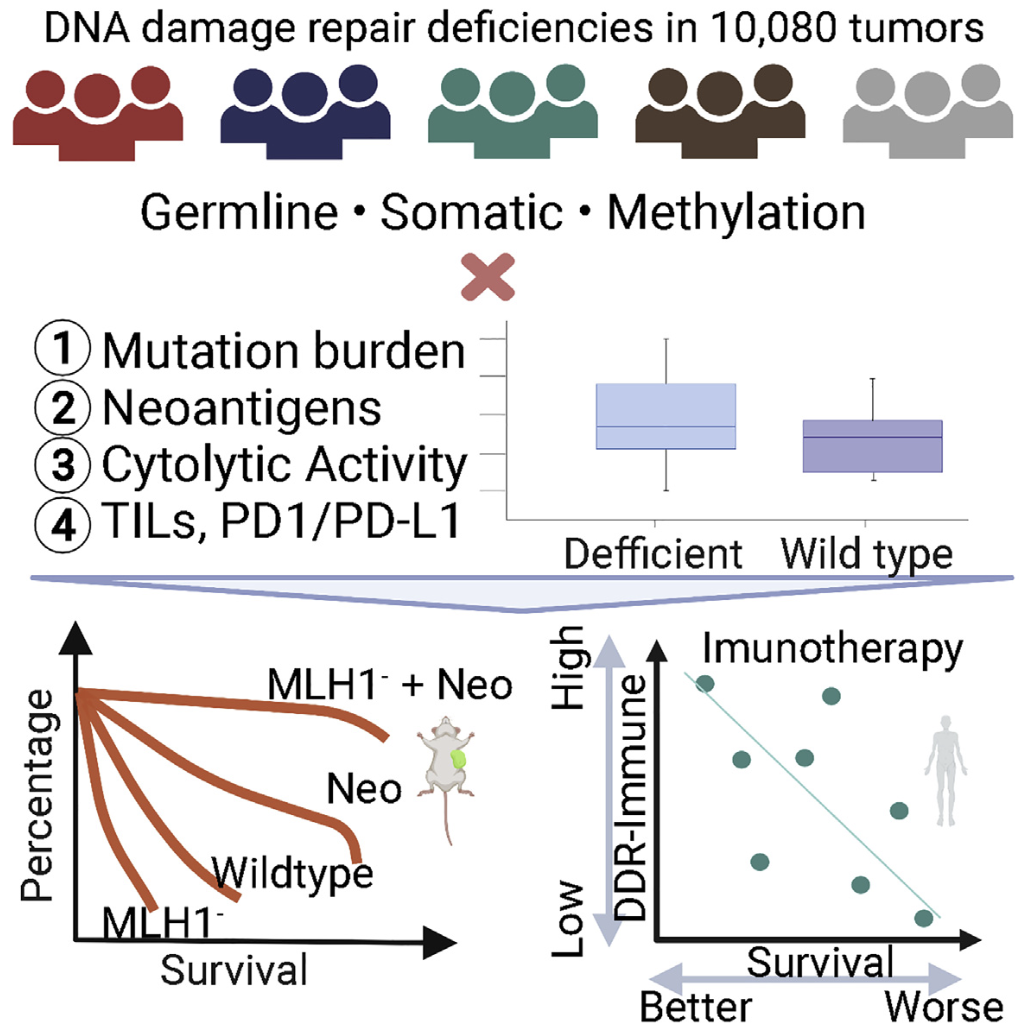
What’s missing, and what we are working on?
To predict disease risk or treatment, the current precision medicine paradigm often relies on single genetic mutations/markers, thus disregarding much of the useful genomic information. Using national/Mount Sinai biobank-scale genomic cohorts, we are actively developing Machine-Learning (ML) models that can more accurately predict disease risks and treatments using the full genome profile, with a focus on incorporating rare and structural variants (SV) in diverse populations.
With 3 billion base pairs of DNA in a genome, it is difficult to identify critical genetic variants in complex diseases. We have effectively prioritized variants by resolving their downstream consequences on the transcriptome, proteome, and structures in cellular, spatial, and longitudinal resolution, with strong interests in protein targets directly related to functions and druggability.
While genes identified from large-scale genomic cohorts can enrich for drug targets, most would still fail in pre-clinical development or clinical trials. We have developed methods to triage the target gene space by integrating multi-omics—in particular those from reverse genetics screens—thus achieving 10X improvement in pinpointing druggable genes.
Acknowledgment
We dedicate our work to study participants, patients, and their family. We thank the health workers at ISMMS and all institutions. And our research would not be possible without funding from these sources:

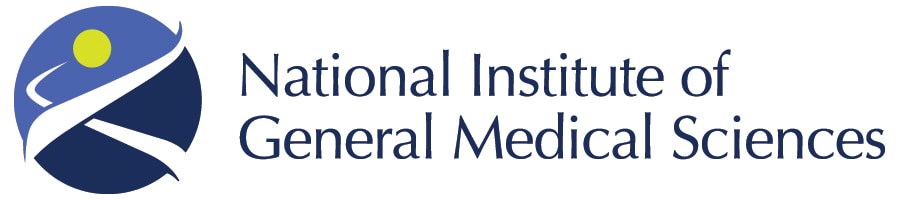
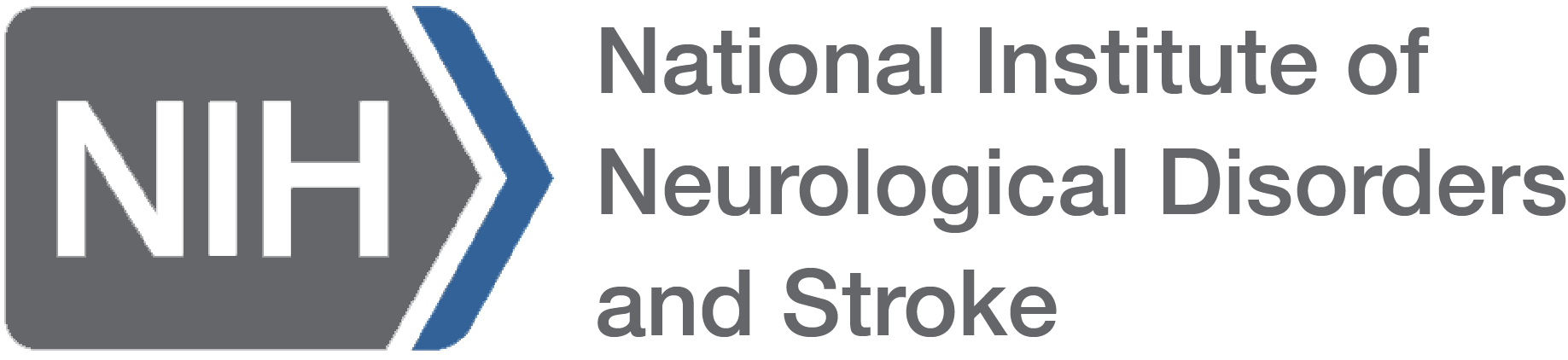
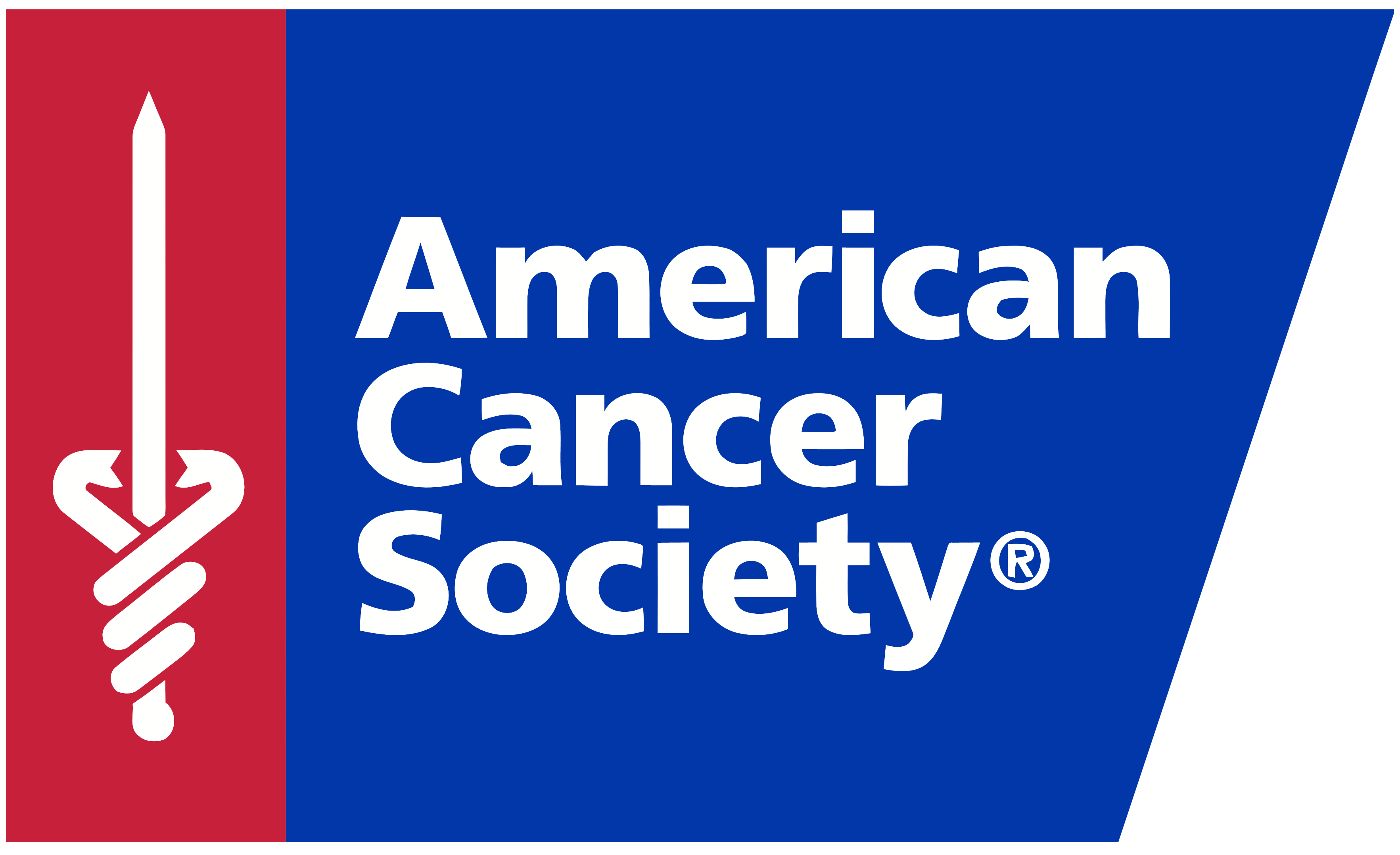
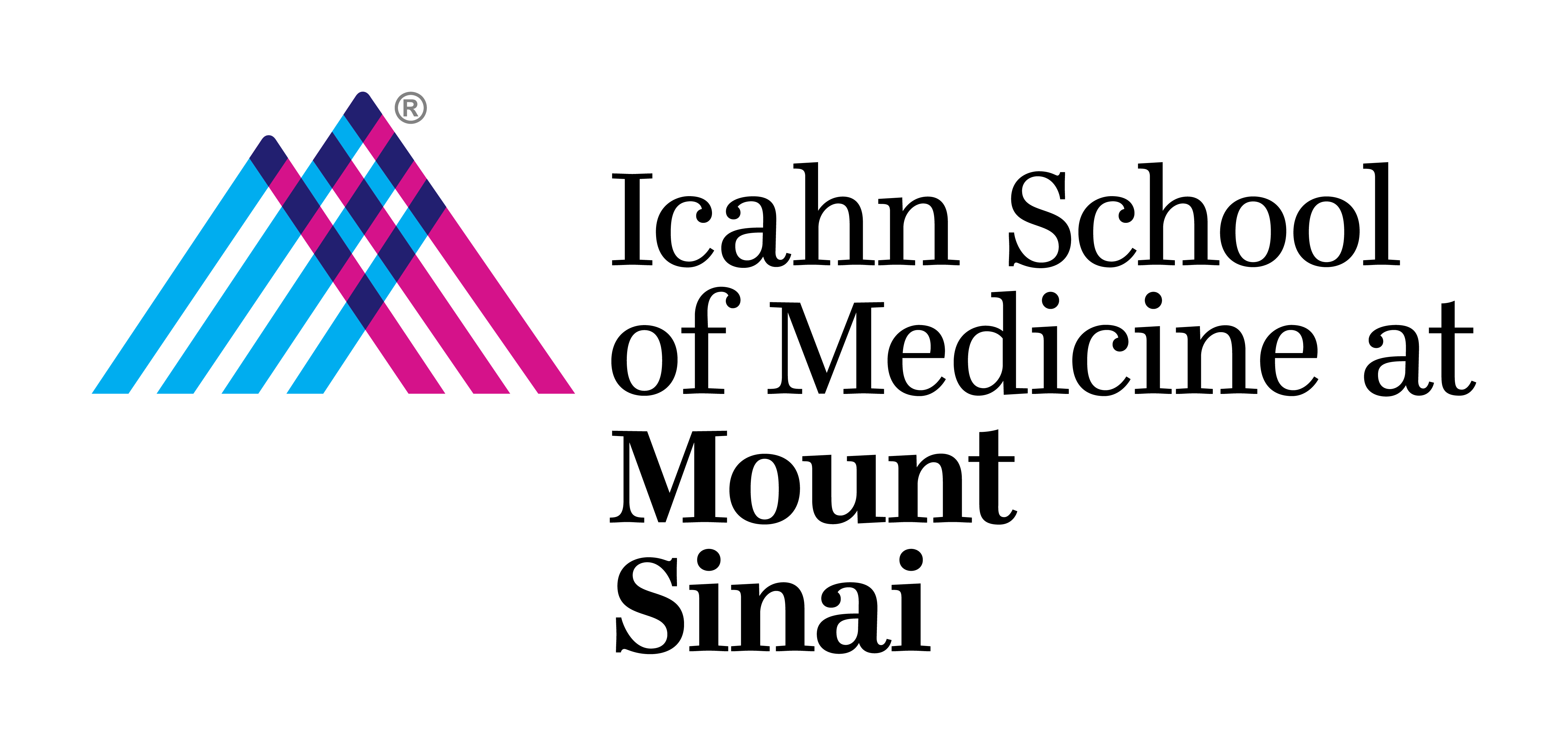
*The top figure includes adopted icons created by Freepik, eotatah, and dDara – Flaticon.