Variant and Genome Interpretation
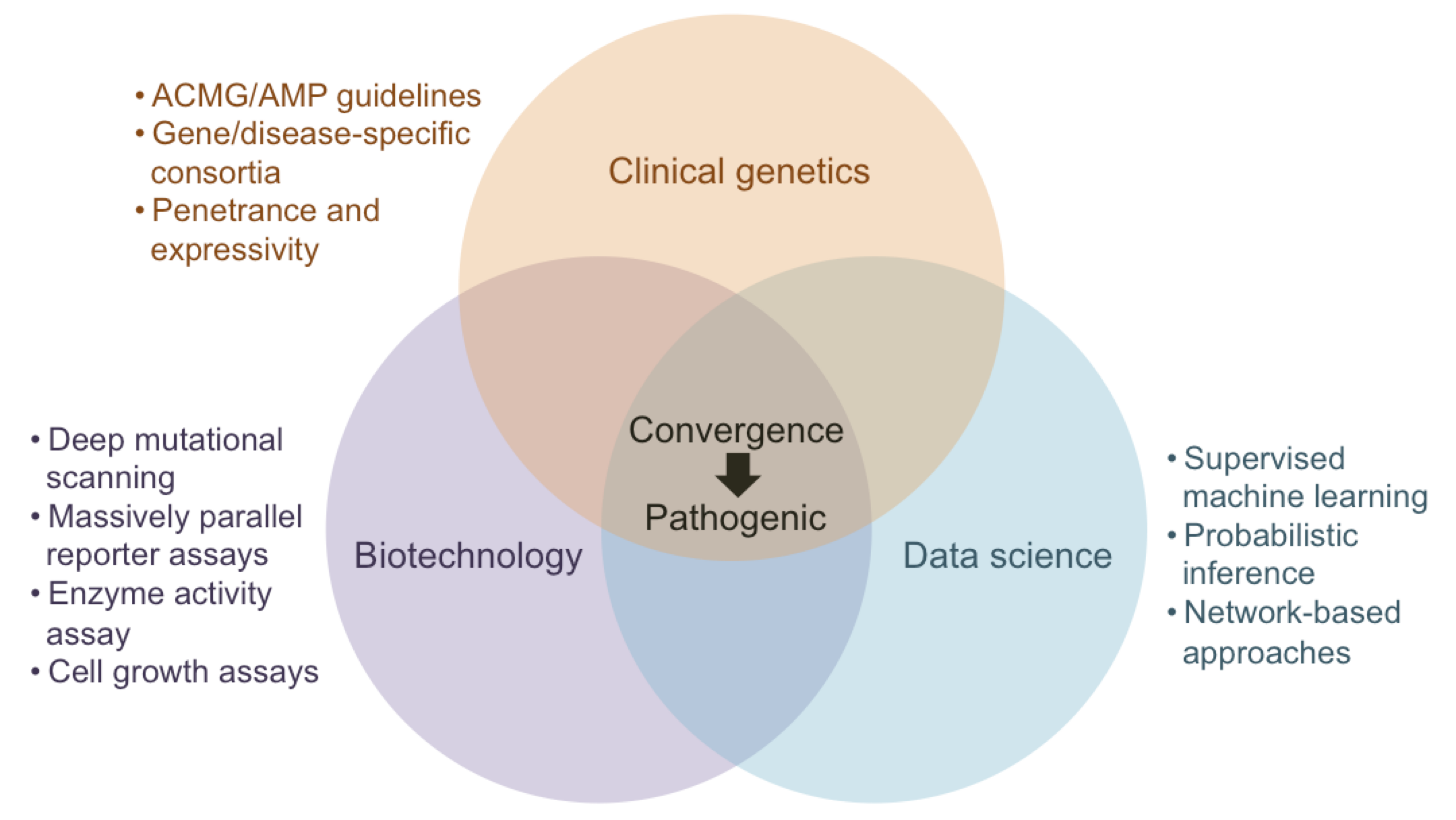
The impact of a single genetic variant can be viewed as a cascade of disruptions propagating through the different levels of a biological system: DNA, RNA, protein, metabolite, pathways, organelle, cell, tissue, organ, organ system. These disruptions, in turn, lead to dysfunction at one or more of these levels that manifest individually or cumulatively at the organismal level as observable changes, i.e., phenotypes. A detailed understanding of how genetic variants lead to human disease has remained one of the grand challenges in the biomedical sciences since the discovery of the first disease-causing variant over 60 years ago. Our research aims to accelerate the formation of this mechanistic understanding of genetic diseases through the development of machine learning models at these different levels. In particular, we focus on the prioritization of impactful genetic variants and the generation of testable hypotheses on their molecular mechanisms. We have previously developed approaches that replace data from heterogeneous sources with machine learning models trained on them and integrate them to make predictions for outcomes that have little to no training data. We have shown empirically that such explicitly modeled features can serve as effective proxies for missing data, help alleviate biases, quantify uncertainty, and engineer novel biologically meaningful features that can explain model predictions. Recently, as affiliates of the Impact of Genomic Variation on Function (IGVF) and the ClinGen Consortium, we have become interested in the convergence of the different sources of information that are used to make a variant “pathogenic” and working towards systematically query and integrate them.
Data-driven Phenotyping
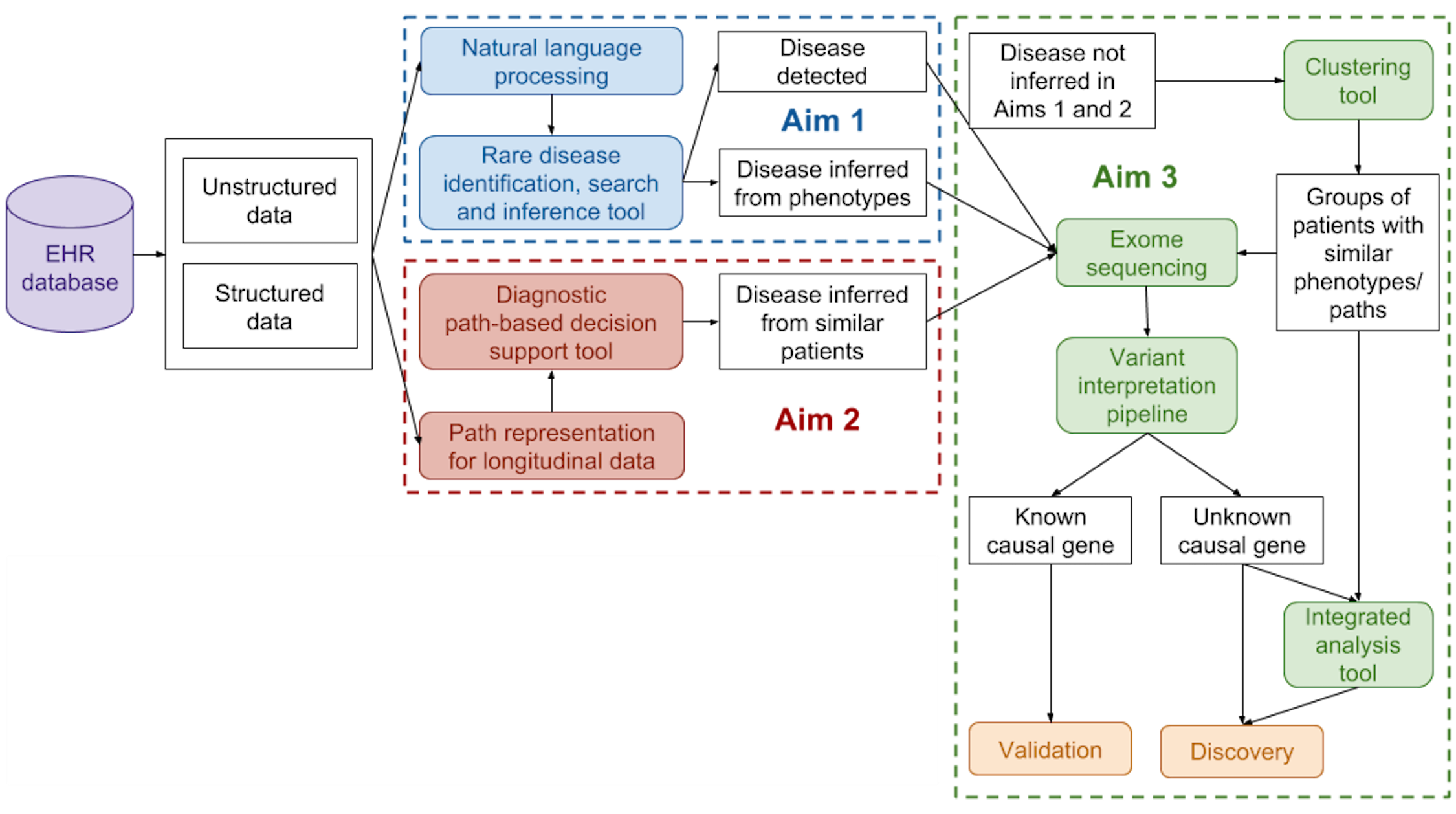
From the perspective of the discovery of causal variants and clinical interpretation of genetic variation, a disease can loosely be defined as groups of phenotypes (at all levels of the biological system) and their patterns of occurrence. While this is a broad and oversimplified definition, it has proven to be powerful in practice, particularly for cohort construction for genome-wide association studies for common diseases and disease delineation in rare genetic disorders. Our lab aims to develop methods to obtain a more precise, data-driven description of disease phenotypes, through the integration of genomic, molecular, and clinical data. We are particularly interested in the joint discovery and interpretation of genetic variants and disease sub-types. Currently, funded projects in the lab include the identification of patients with rare genetic disorders from their health records (particularly from unstructured clinical notes using natural language processing methods), the development of phenotypic risk scores for hereditary transthyretin amyloidosis (hATTR), and familial hypercholesterolemia.
Applied Machine Learning
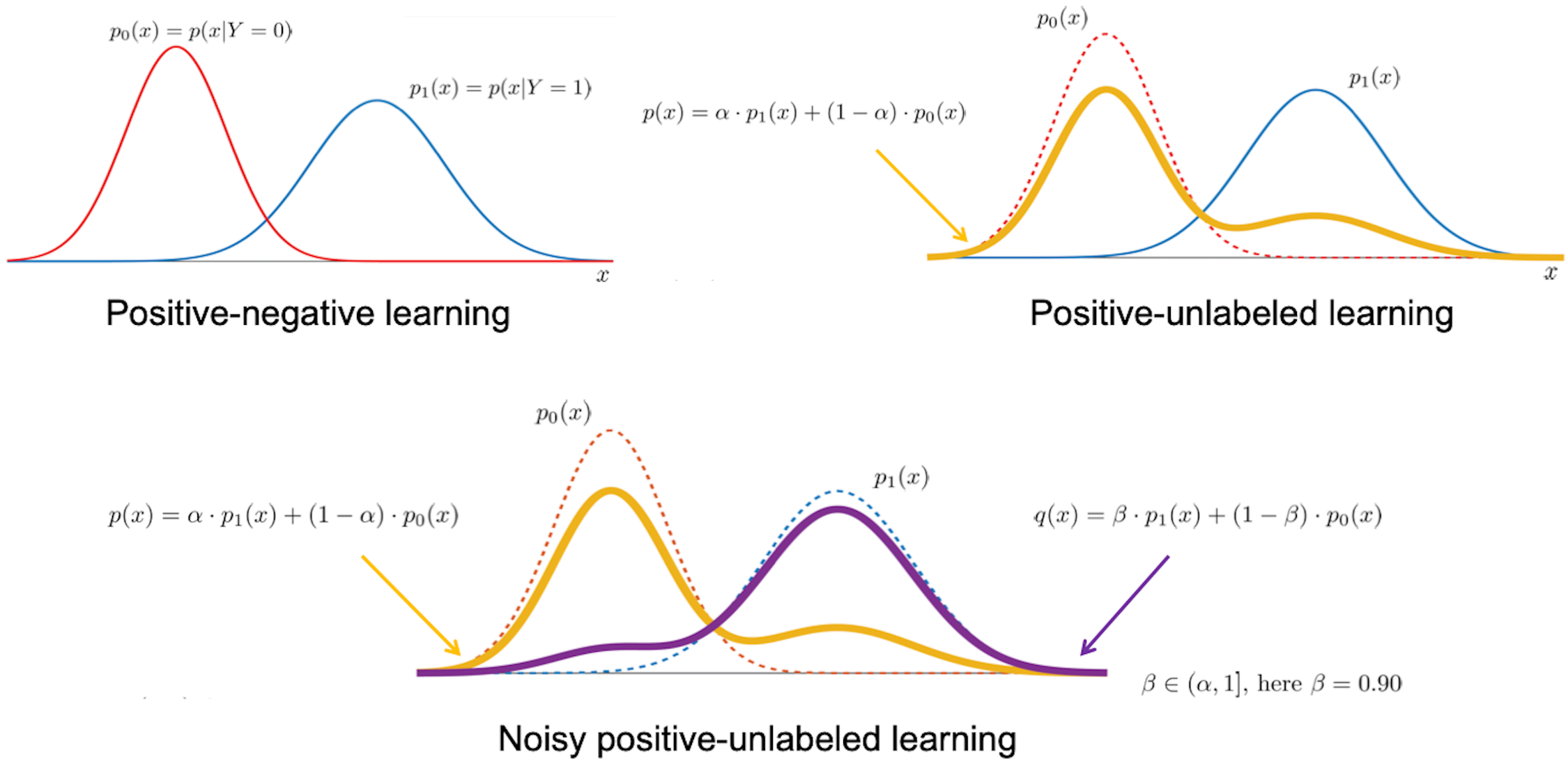
Our biomedical research interests naturally lend themselves to the development of innovative problem formulations and the adaptation of modern concepts in machine learning. While our previous focus has largely been on recasting typical prediction problems in biomedicine in a noisy positive-unlabeled learning framework, we are particularly interested in techniques to learn from text data, explain machine learning predictions, and those in structured output learning, active learning and similarity learning. We also take a keen interest in the development of novel metrics to evaluate machine learning methods for biomedical data sets. We are particularly interested in the tradeoffs between typical metrics in machine learning and those that influence decision-making in experimental design and clinical settings. We have actively served as participants and assessors in Critical Assessment of Genome Interpretation (CAGI), a community-wide experiment to evaluate variant and genome interpretation methods. In collaboration with the ClinGen Consortium, we are working on updated recommendations for the use of computational tools in clinical variant classification.