Tisch Cancer Institute (TCI) Biostatistics Shared Resource (BSR)
Welcome to the TCI-BSR website. We have wide-ranging expertise in biostatistical and clinical informatics methods and also provide training and educational resources.
Requesting Services? click here
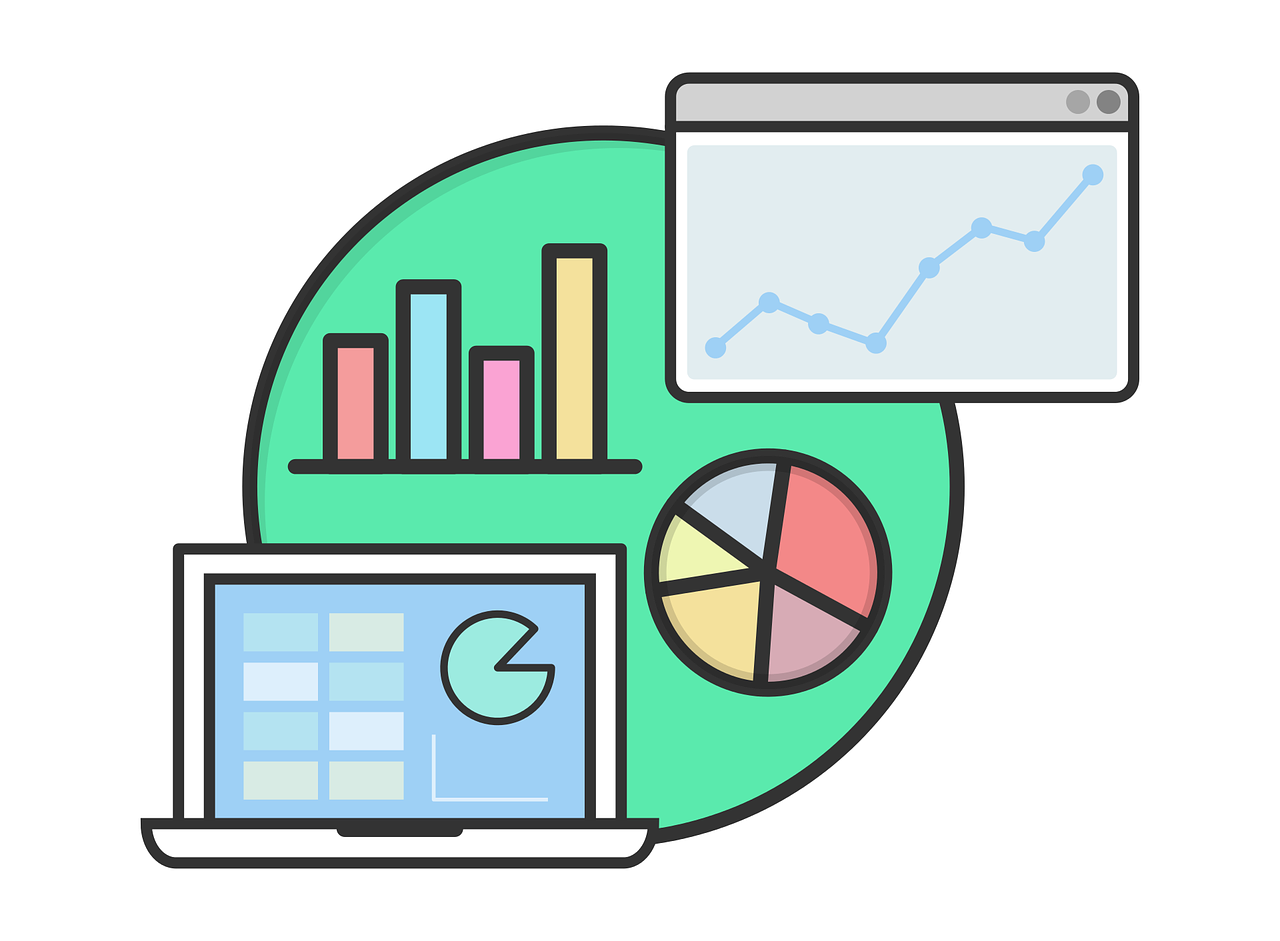
Biostatistics
Clinical Informatics
Gathering and synthesizing large amounts of cancer data from multiple sources, as well as efficiently implementing such information into clinical practice has become increasingly important. The multiple domains of data in the cancer control continuum frequently raises complex challenges in data management, integration, and analysis. Data scientists and informaticians of many stripes play a key role in these efforts. Successful internal and external collaboration is often required for high-impact publications, grantsmanship, and advances in clinical care.
Our clinical informatics experts support clinical, translational, and basic science TCI investigators by enhancing and extending Mount Sinai’s informatics infrastructure to make cancer research data and software tools easily accessible. In addition, we aim to establish clinical informatics as an academic discipline at TCI and foster national collaborations to accelerate informatics ideas, best practices, technologies, and standards.
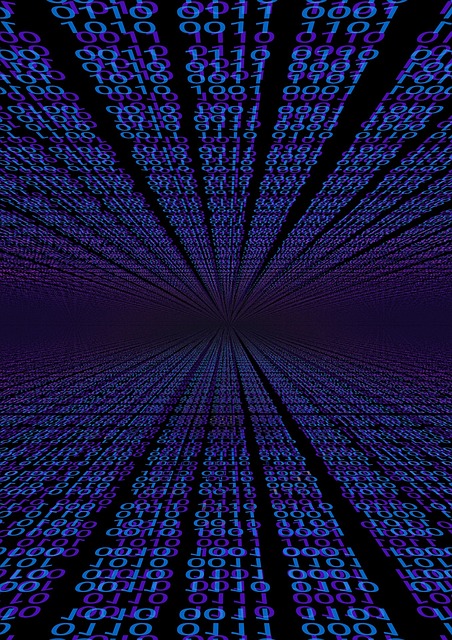
Biostatistics core utilizes Bayesian Optimal Interval Phase I/II (BOIN12) design to assess safety and efficacy of a combination regimen for myelodysplastic syndrome/myeloproliferative neoplasm (MDS/MPN) overlap syndrome
For many novel therapies where the efficacy does not necessarily increase with the dose, the maximum tolerated dose (MTD) may not be the optimal dose for treating patients and the goals of dose-finding trials needs to be identifying the optimal biologic dose (OBD) that optimizes patients’ risk-benefit trade-off. A flexible Bayesian optimal interval phase I/II (BOIN12) design is introduced to find that OBD. This design makes the decision of dose escalation and de-escalation by simultaneously taking account of efficacy and toxicity and adaptively allocating patients to the dose that optimizes the toxicity-efficacy trade-off. Our physician investigators found this design to be simpler to comprehend and implement because it overcomes the computational and implementation complexity that plagues existing Bayesian phase I/II dose-finding designs.
Story 2 - Erin's complicated data analysis
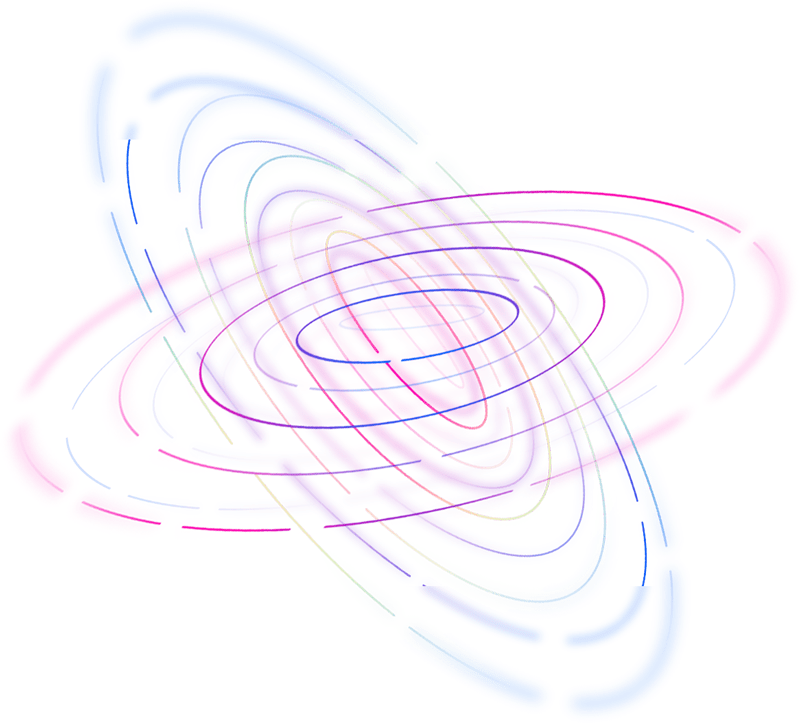
Clinical informatics facilitates risk prediction to guide personalized bladder cancer treatment decisions
Clinical guidelines support the use of neoadjuvant chemotherapy (NAC) for the treatment of muscle-invasive bladder cancer (MIBC), but NAC remains used in only a small subset of patients. An important barrier is the difficulty in predicting and communicating individual survival estimates with or without NAC. Through a collaboration of clinical and qualitative researchers, we developed individualized risk prediction and communication methods to facilitate NAC decision making using the National Cancer Database. Based on state-transition modeling, five-year cancer mortality and other-cause mortality risk estimates were generated and integrated within a web-based tool. Clinicians thought the prediction tool was “easy to use” (100%) and would use it “frequently in their practice” (92%).
Clinical informatics with MSHS data science team develops a malnutrition screening tool to increase diagnosis rate
The Joint Commission has mandated universal screening and assessment of hospitalized patients for malnutrition because of its association with increased morbidity, mortality, and healthcare costs. Early detection is extremely important for timely intervention but difficult to achieve due to need for specialized skills from registered dieticians (RDs). Machine learning (ML) algorithms, which process massive amounts of data and learn continuously have the potential to identify those at risk for malnutrition more efficiently and effectively than standard screening tools. A clinical decision support tool, MUST-Plus, developed by this team reduced lag between admission and diagnosis, had high usability (> 90%), and increased the rate of malnutrition diagnoses and its documentation.