Welcome To Our Lab
Our lab develops statistical and computational approaches (see Software) to understand how human genetic variation, in combination with the environment, leads to disease. Given their proxy for genetic liability itself, our primary focus is on the theory and application of Polygenic Risk Scores (PRS). In 2015, we published the popular PRS software, PRSice (‘precise’), followed in 2019 by PRSice-2 (PRSice website here). We have run several PRS workshops (eg. our PRS Summer School) and in 2020 we published our Guide to PRS paper, with accompanying PRS tutorial.
We believe that genetic liability to disease is more complex than implied by the additive model of present polygenic risk scores, that the interplay between the genome and the environment in causing disease needs to be better understood, and that analysis of diverse populations across diverse environments can provide the greatest power to understand the causes of disease.
The research in our lab follows 4 key themes:
1) Pathway-specific, function-informed, polygenic risk scores
2) Polygenic risk scores for diverse and admixed populations
3) Using genetics to infer the environmental causes of disease
4) The Statistical Genetics of Brain Disorders
We need to bring together the fields of statistical genetics, GWAS, functional genomics, population genetics and epidemiology in order to understand how individual genetic profiles combine with the environment to produce human traits and disease – so if you are interested in any of these fields and the research of our lab then please feel free to email Paul (paul.oreilly@mssm.edu) to discuss more or to enquire about our open student and postdoc positions.
Contact Us
O’Reilly Lab
Location: Department of Genetics and Genomic Sciences, Icahn School of Medicine, Icahn School of Medicine at Mount Sinai, New York, New York
Email: paul.oreilly@mssm.edu
Lab Themes
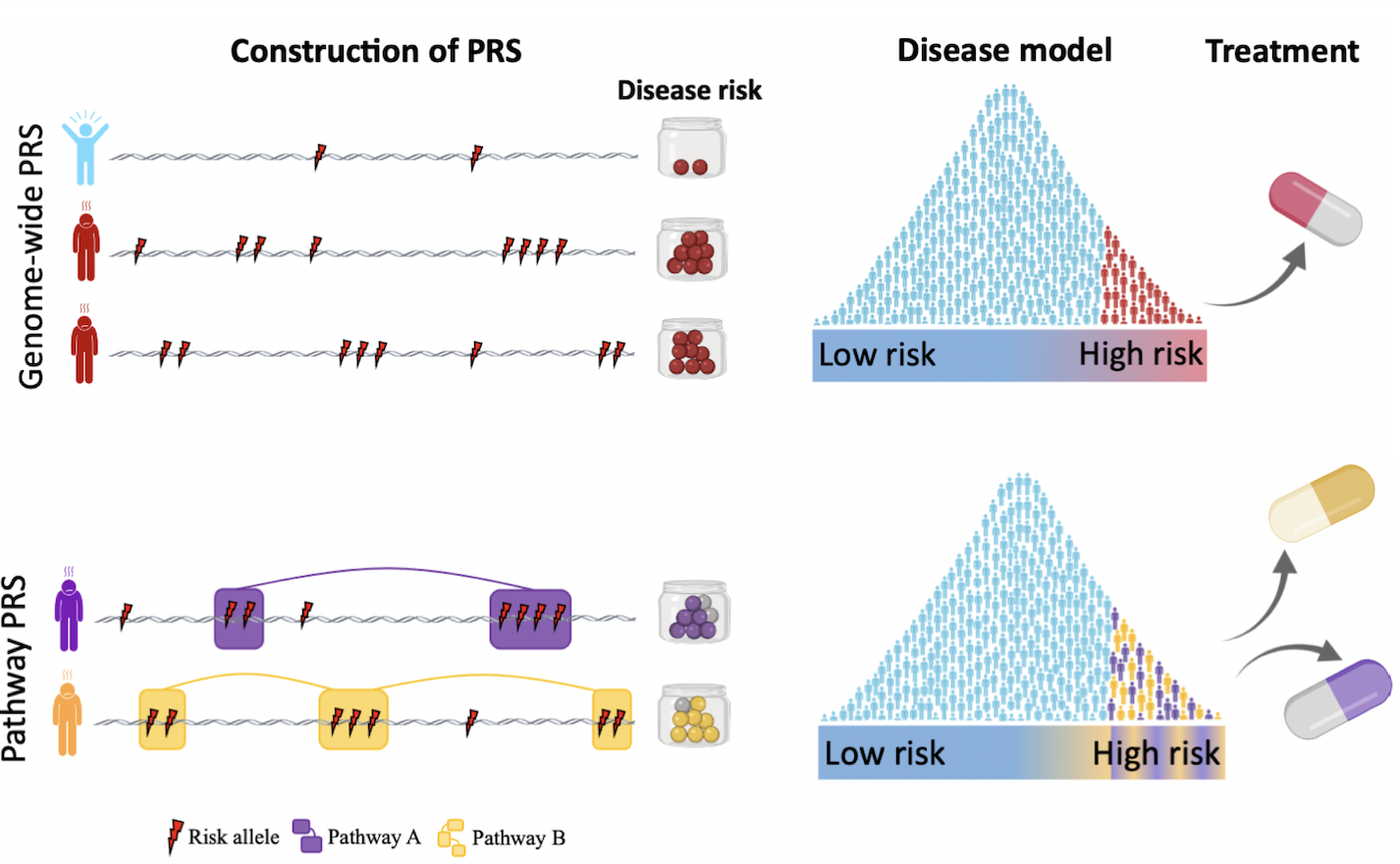
Pathway-specific, function-informed, PRS
The standard polygenic model, which assumes that everyone lies on a linear spectrum from low to high risk, is an over-simplification given the known heterogeneity of disease and functional sub-structure of the genome. A primary focus in our lab is on developing pathway-specific, function (i.e. multi-omics) informed, polygenic risk scores. We believe that these will better reflect how genetic liability manifests and leads to disease, as compared to standard PRS that only consider genome-wide aggregated risk irrespective of specific genetic profiles. Moreover, we believe that pathway-specific PRS will pave a clearer path towards stratified medicine given their focus on functional variability of individual genetic profiles.
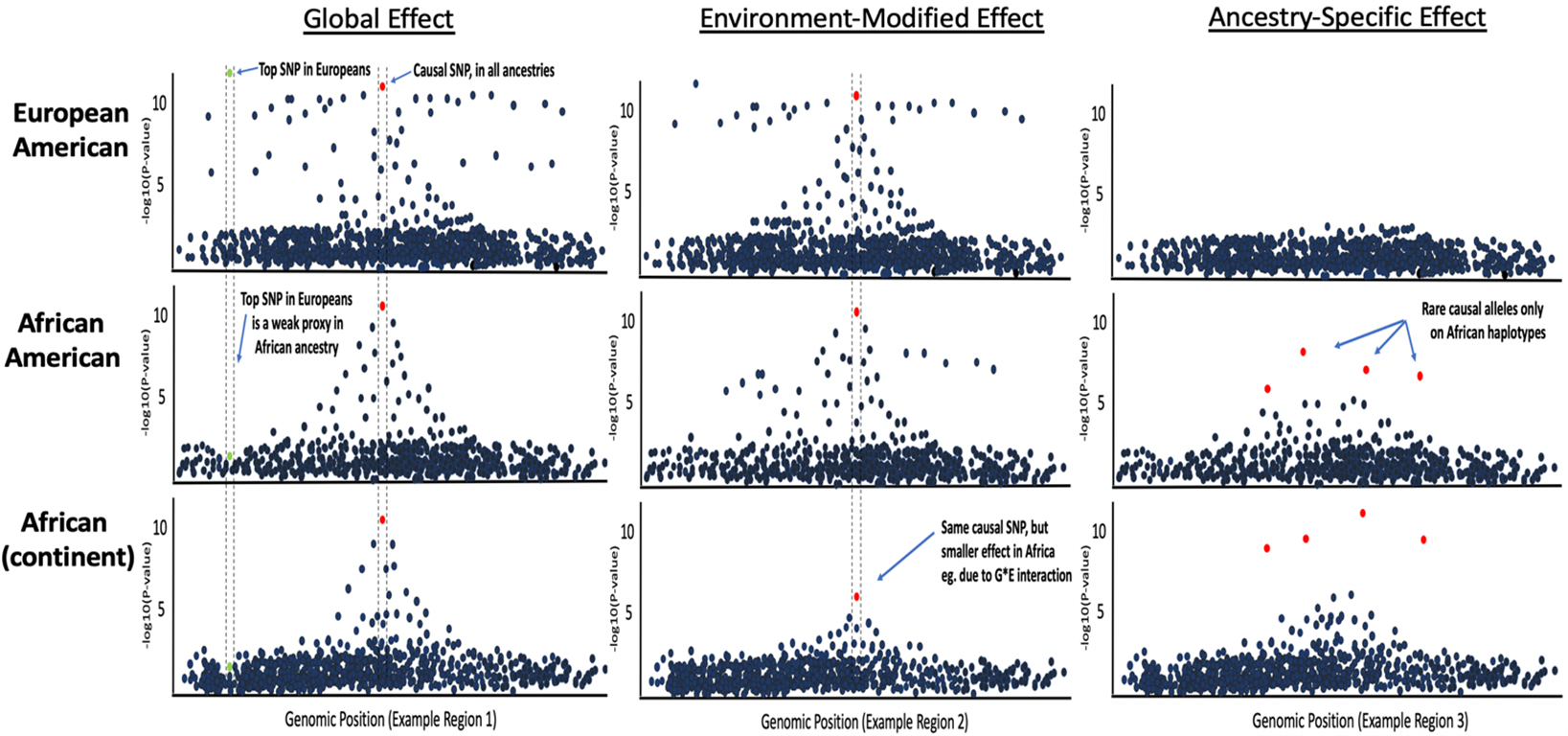
PRS for diverse and admixed populations
PRS are mostly derived from European-ancestry GWAS, making their predictive power lower when computed in individuals of non-European ancestry. This problem has been widely reported in recent years, yet PRS in e.g. recent African-ancestry individuals are still often computed using European GWAS and PRS methods applied to European data. This takes no account for known population genetic factors affecting the data, such as: LD, genetic drift, natural selection and G*E interactions. The clinical utility and aetiological insights provided by PRS may have limited relevance to individuals of recent African and other non-European ancestry unless PRS methods are developed specifically for application to diverse and admixed populations. Moreover, performing research in diverse populations living in diverse locations is an ideal way to better understand the genetic and environmental causes of disease.
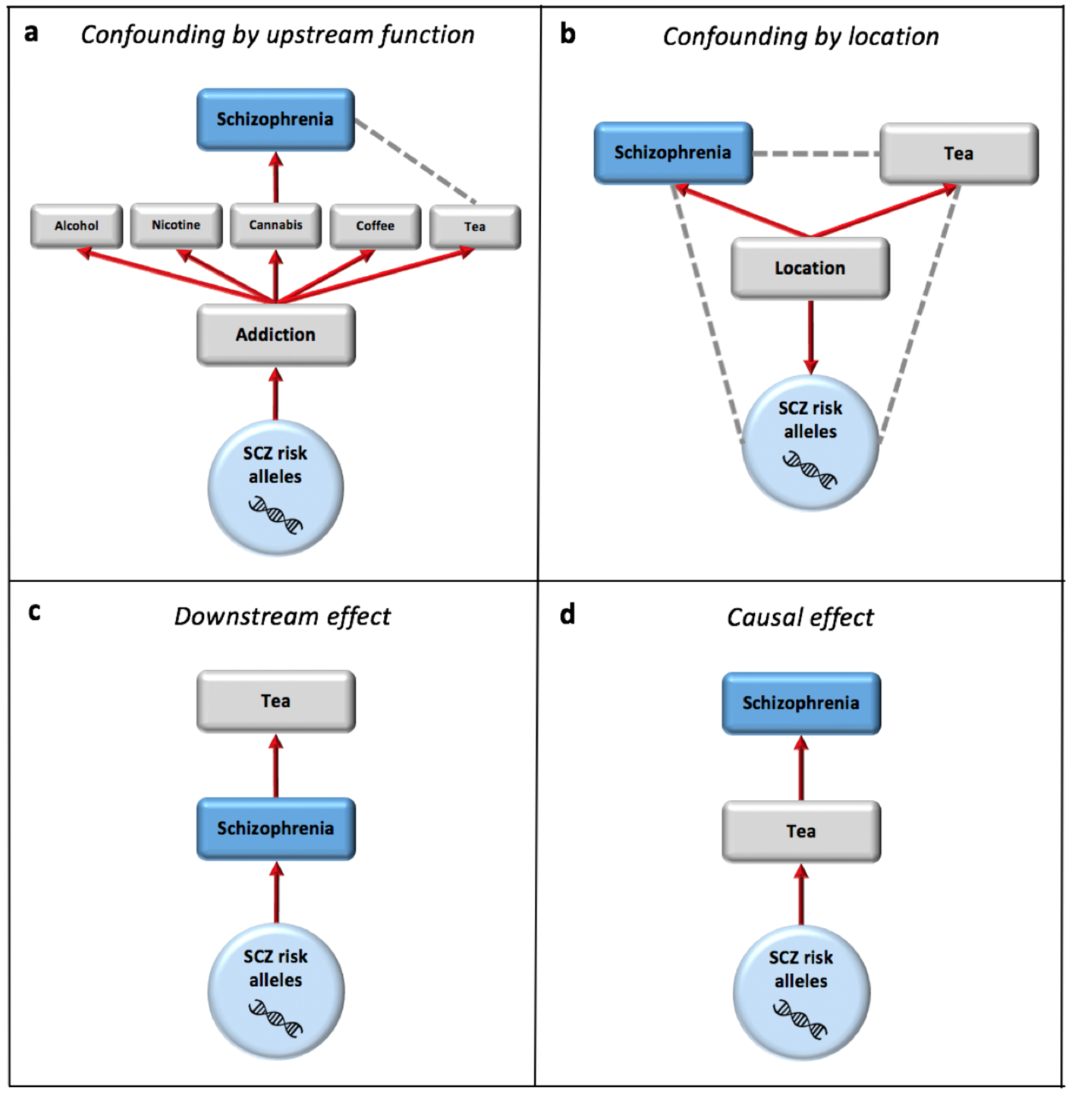
Using genetics to infer the environmental causes of disease
Almost all environmental risk factors for disease have a genetic component, which itself must be a genetic component of the disease. This creates a complex interplay between genetic and environmental causes of disease, which needs to be investigated carefully in order to disentangle the effects of each. While genetic variants that are convincingly associated with disease (controlling for pop. structure) have the convenient feature that they must be causal, they may only be causal because of environmental risk factors that they interact with or trigger, and so could be non-causal in other environmental settings or if the environment changes (e.g. due to health policy intervention or social changes). However, because of this, genetics provides an opportunity to test the causality between putative environmental risk factors and disease – but only if the complex network between genetics and the environment (both cellular and society level) is accounted for and accurately modelled.
Team
Paul O’Reilly
I am an Associate Professor in Statistical Genetics at the Icahn School of Medicine at Mount Sinai NYC, having joined in 2019 from King’s College London (previously at Imperial College London, where I did my PhD supervised by David Balding and where I became faculty in 2011). In order to gain insights into how the human genome evolves and gives rise to disease, I have developed Statistical Genetics methods and software in Genetic Epidemiology (eg. multi-trait GWAS: MultiPhen, Polygenic Risk Scores: PRSice) and Population Genetics (eg. detecting selection: Ped/Pop method, simulating inversions: invertFREGENE), while my applied work focuses on the statistical genetics of brain disorders.
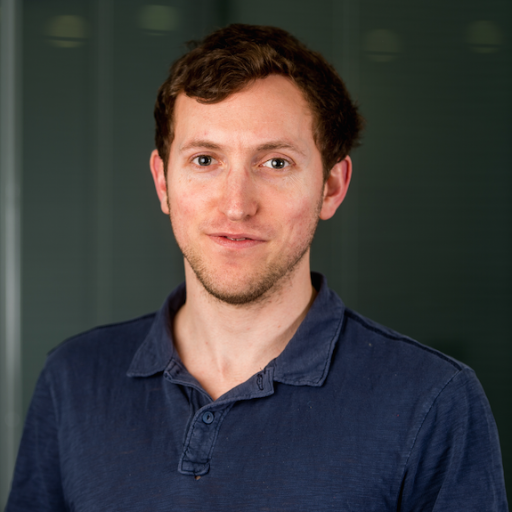
Email: paul.oreilly@mssm.edu
Beatrice Wu
I am a computional scientist focused on the application of statistical genetics methods and software to brain disorders, in particular schizophrenia and Alzheimer’s disease. My PhD focused on identifying genetic risk factors of schizophrenia, supervised by Pak Sham at the University of Hong Kong. I joined the O’Reilly in 2016 at King’s College London and have since focused on the application of polygenic scores to psychiatric disorders and Alzheimer’s disease.
Clive Hoggart
I am a statistician focused on Bayesian approaches and predictive modelling in statistical genetics, having completed a PhD in Bayesian methods in forensic science supervised by Adrian Smith. I have produced numerous methods in statistical genetics, including methods for admixture mapping (including software ADMIXMAP), novel methodology and software for the joint modelling of all SNPs genome-wide in genetic association studies (HyperLASSO), estimation of missing heritability attributable to allelic heterogeneity and identification of parent-of-origin effects using genetic data from unrelated individuals. Since moving to the Icahn School of Medicine in 2020, my research focus has been on methods to improve the portability of polygenic risk scores across different populations and ancestries.
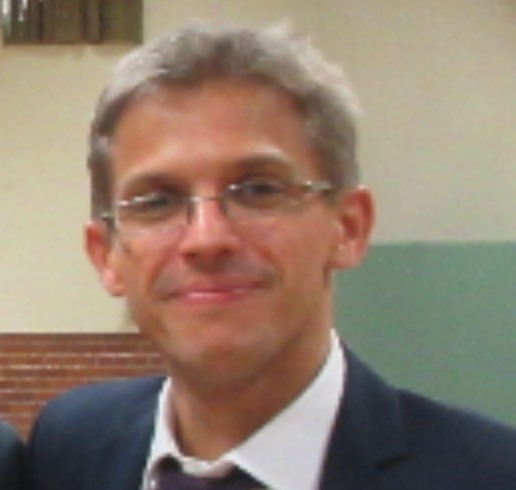
Email: clive.hoggart@mssm.edu
Conrad Iyegbe
I have core training in molecular and quantitative genetic analysis from King’s College London (PhD in Genetics) and postdoctoral research training in psychiatric research. My previous research interests combine methods development with a focused interest in understanding how gene-environment interplay shapes risk for disease at statistical and functional levels. I currently work on strategies to improve polygenic risk score performance across global populations. I co-lead an annual training workshop on polygenic risk score analysis, run in conjunction with Wellcome Connecting Science. As part of my commitment to improving health outcomes on a global level I co-chair the Africa division of the Psychiatric Genomics Consortium which was created in September 2022 to implement a strategic agenda for psychiatric genomics research in Africa.
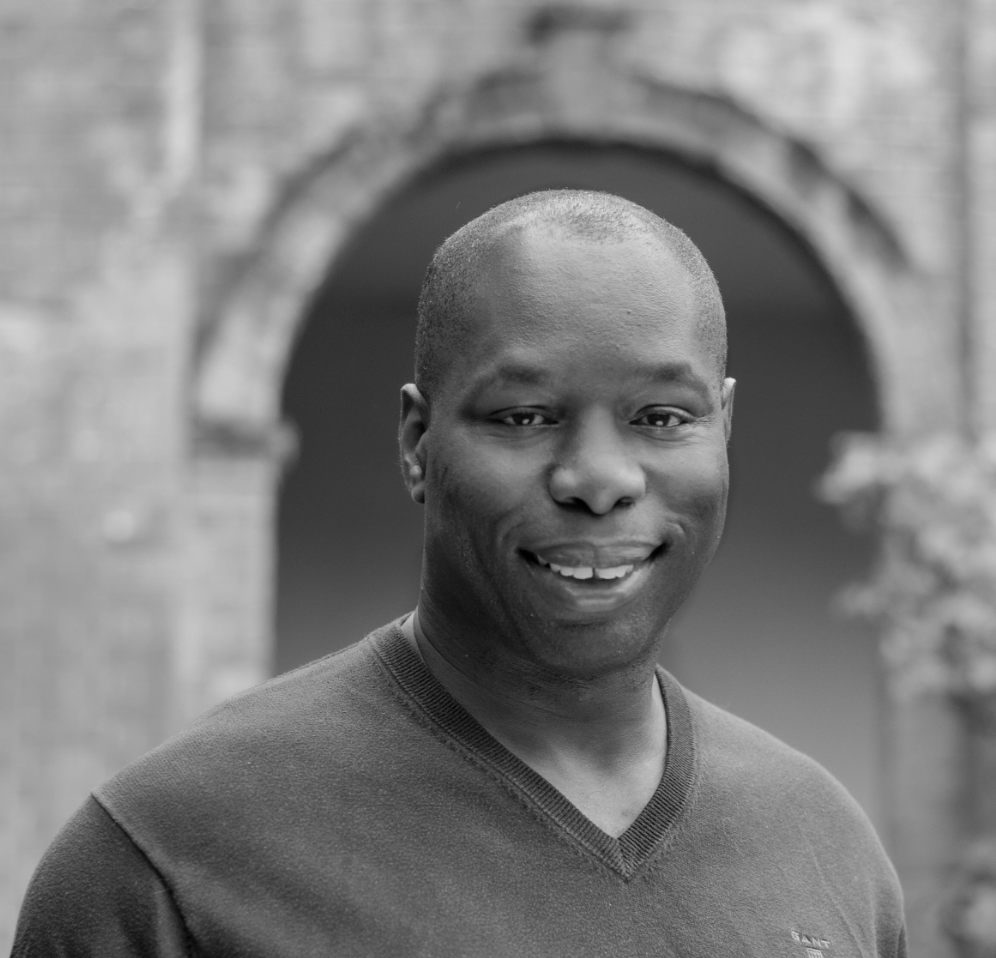
Email: conrad.iyegbe@mssm.edu
Judit García-González
I am a computational biologist with a background in experimental and statistical genetics research. I received my PhD in genetics from Queen Mary University of London, where I investigated the interplay between smoking and genetic vulnerability to psychiatric disorders using animal models and statistical genetics approaches in human cohorts. In late 2020, I joined the O’Reilly lab to develop methods that incorporate functional genomic information to polygenic risk scores, with a focus on psychiatric disorders.
Anil Ori
As a scientist, my work is motivated by a commitment to improving health
outcomes and addressing health disparities. I specialize broadly in
medical and population genomics, and health equity. I have joined the
Icahn School of Medicine at Mount Sinai NYC and the PRS lab in the
summer of 2022 and am currently investigating how evolutionary genetic
processes, such as selection, can help us understand the genetic
architecture of complex traits. This is important as a better understanding
of trait architecture can for example improve downstream genetic
analyses, such as gene discovery and PRS predictions. Additionally, I am
affiliated with the Department of Psychiatry at the University Amsterdam
Medical Center in the Netherlands, where I co-lead a recently funded
project measuring population representation, specifically in terms of
racial/ethnic composition, across medical cohorts in the Netherlands
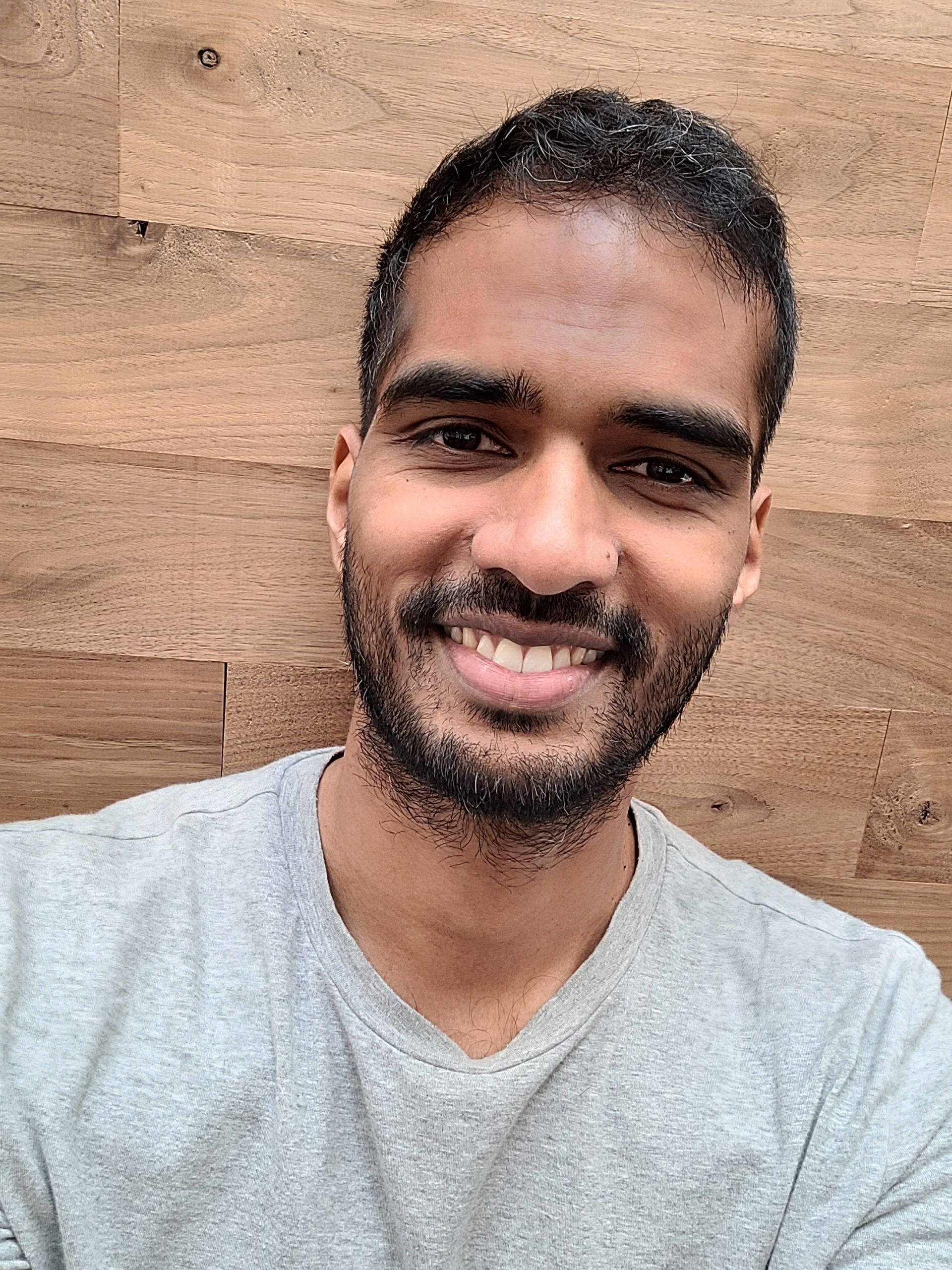
Email: anil.ori@mssm.edu
Twitter: @anilpsori
Lathan Liou
I am a medical student at the Icahn School of Medicine at Mount Sinai. I received my M.Phil. in genetic epidemiology from the University of Cambridge, where I studied the application of cardiovascular polygenic risk scores to cancer cohorts under the supervision of Paul Pharoah. I stayed to work at the MRC Biostatistics Unit where I helped develop the onlineFDR framework with David Robertson. I then worked as a data scientist at Merck. I joined the O’Reilly Lab to study how to apply statistical genetics to improve clinical decision-making.
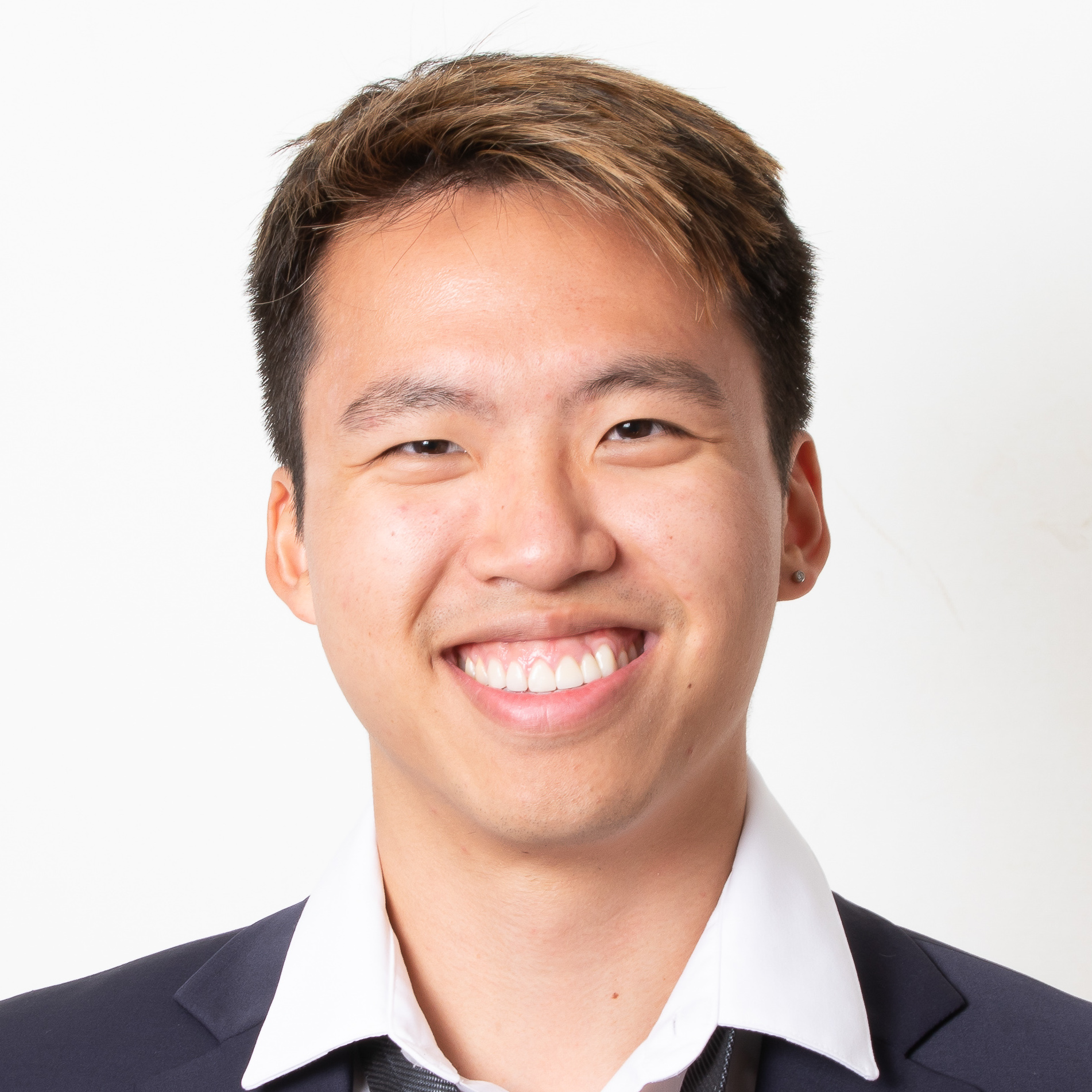
Email: lathan.liou@icahn.mssm.edu
Alanna Cote
I am a computational researcher with a background in functional genomics and transcriptomics of brain disorders. I completed my PhD at the Icahn School of Medicine supervised by Dr. Laura Huckins, with a focus on quantifying artifact- and genotype- driven patterns of co-expression in the prefrontal cortex. I joined the O’Reilly Lab in early 2024, where my focus has been on developing methods to incorporate functional information in pathway-specific PRS.
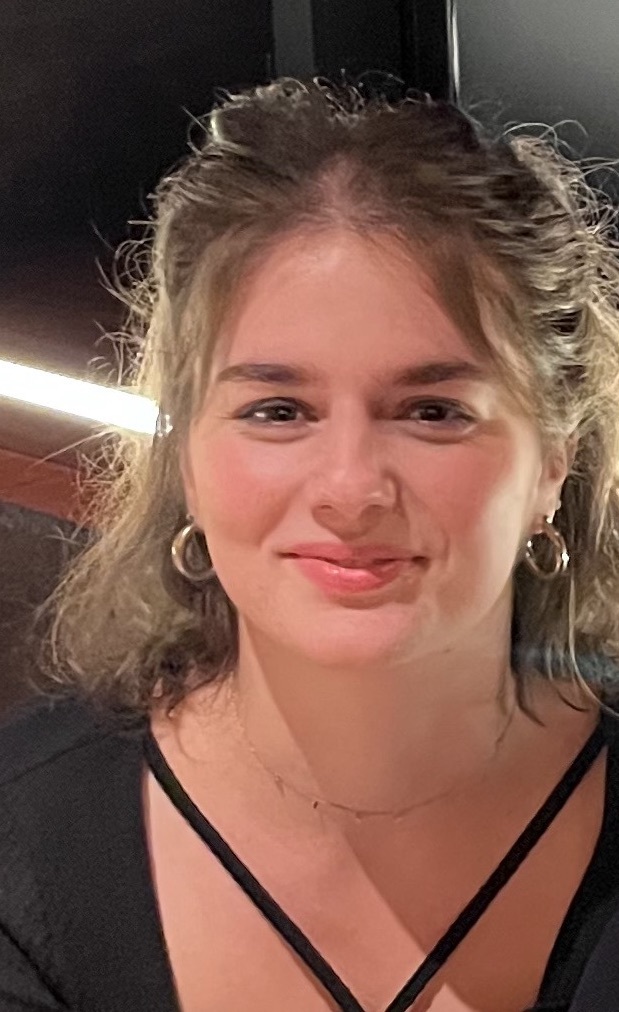
Email: alanna.cote@icahn.mssm.edu
Twitter: @AlannaCote2
Selected Publications
Hoggart CJ,…, O’Reilly PF. 2023. Nature Genetics.
Privé F,…, O’Reilly PF, Vilhjálmsson BJ. 2022. Am J Hum Genet.
EraSOR: a software tool to eliminate inflation caused by sample overlap in polygenic score analyses
Choi SW, Mak TS, Hoggart CJ, O’Reilly PF. 2023. Gigascience.
PRSet: Pathway-based polygenic risk score analyses and software
Choi SW*, García-González J*…,O’Reilly PF. 2023. Plos Genetics.
Corte L, Liou L, O’Reilly PF, Garcia-Gonzalez J. 2023. Gigabyte.
Sibling Similarity Can Reveal Key Insights Into Genetic Architecture
Souaiaia, T, Wu HM, Hoggart CJ, O’Reilly PF. 2023. eLife.
Heterogeneous effects of genetic risk for Alzheimer’s disease on the phenome
Wu HM, Goate A, O’Reilly. 2021. Translational Psychiatry.
Investigating the effects of genetic risk of schizophrenia on behavioural traits
Socrates A,…, O’Reilly PF. 2021. NPJ Schizophrenia.
Tutorial: a Guide to Performing Polygenic Risk Score Analyses
Choi SW, Mak TS, O’Reilly PF. 2020. Nature Protocols
PRSice-2: Polygenic Risk Score Software for large-scale data
Choi SW & O’Reilly PF. 2019. GigaScience
García-González J et al. 2020. Translational Psychiatry
Using genetic data to strengthen causal inference in observational research
Pingualt JB, O’Reilly PF et al. 2018. Nature Reviews Genetics
The emerging molecular architecture of schizophrenia, polygenic risk scores and the clinical implications for GxE research
Iyegbe C et al. 2014. Social Psych. and Psych. Research
Effect of Damaging Rare Mutations in Synapse-Related Gene Sets on Response to Short-term Antipsychotic Medication in Chinese Patients With Schizophrenia: A Randomized Clinical Trial
Wang Q*, Wu HM* et al. 2018. JAMA Psychiatry
Multivariate simulation framework reveals performance of multi-trait GWAS
Porter HF and O’Reilly PF. 2017. Scientific Reports
MultiPhen: Joint Model of Multiple Phenotypes Can Increase Discovery in GWAS
O’Reilly PF et al. 2012. PLOS ONE
Novel approach identifies SNPs in SLC2A10 and KCNK9 with evidence for parent-of-origin effect on body mass index
Hoggart CJ et al. 2014. PLoS Genetics
Genome-Wide Association Study Identifies Six New Loci Influencing Pulse Pressure and Mean Arterial Pressure
Wain*, Verwoert*, O’Reilly*, Shi*, Johnson* et al. 2011. Nature Genetics
Confounding between recombination and selection, and the Ped/Pop method for detecting selection
O’Reilly PF, Birney E, Balding DJ. 2008. Genome Research
InvertFREGENE: Software for Simulating Inversions in Population Genetic Data
O’Reilly PF, Coin LCM, Hoggart CJ. 2010. Bioinformatics
Admixture provides new insights into recombination
O’Reilly PF & Balding DJ. 2011. Nature Genetics
Fine-scale estimation of location of birth from genome-wide SNP data
Hoggart CJ*, O’Reilly PF* et al. 2012. Genetics
Simultaneous Analysis of all SNPs in Genome-Wide and re–sequencing association studies
Hoggart CJ, Whittaker JC, De Iorio M, Balding DJ. 2008. PLoS Genetics
Software
Methods and software for GWAS and PRS analyses:
- PRSice-2: Software for calculating PRS for large-scale data
- PRSet: Software for calculating and analysing pathway-specific PRS
- BridgePRS: Software for addressing the portability problem in PRS
- EraSOR: Software for removing bias introduced from having overlapped samples between base GWAS and target genotype data.
- Trumpet Plots: Software for visualizing the relationship between allele frequency and effect sizes in GWAS
- MultiPhen: Method and R package for performing multi-trait GWAS on individual-level genetic data
- hyperLASSO: Method for performing GWAS on all SNPs genome-wide simultaneously
- ADMIXTUREMAP: Method for performing admixture association studies
Methods and software for population genetic simulation and inference:
- invertFREGENE: Software for simulating inverse polymorphisms in population genetic data.
- pcLOCATE: Method for inferring location of birth from genetic principal componentsd for inferring location of birth from principal components
- Ped/Pop: Method for detecting recent positive selection by comparing pedigree and population recombination rates
- BAYESFST: Bayesian method and software for detecting selection based on the Fst statistic
Open Positions
We need to bring together the fields of statistical genetics, GWAS, functional genomics, population genetics and epidemiology in order to understand how individual genetic profiles combine with the environment to produce human traits and disease. If you are interested in any of the lab themes and the research of our lab then please feel free to email Paul (paul.oreilly@mssm.edu) to discuss more or to enquire about our open student and postdoc positions.