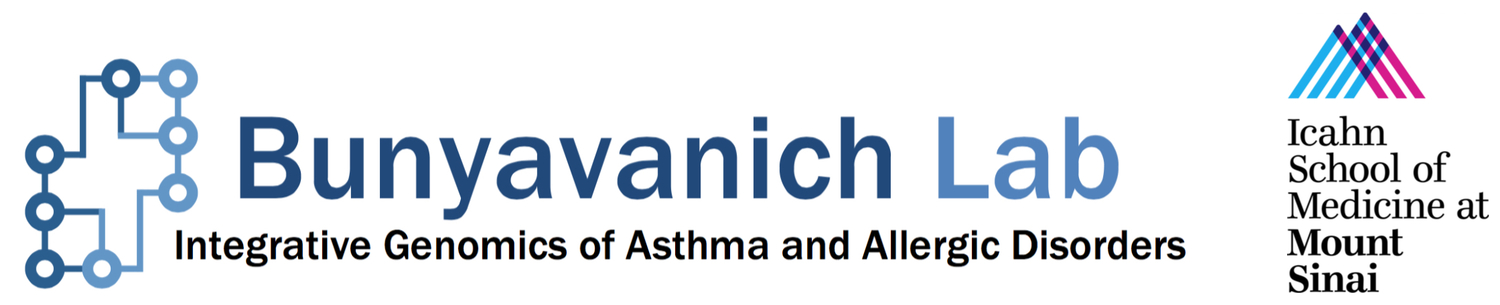
Our Research
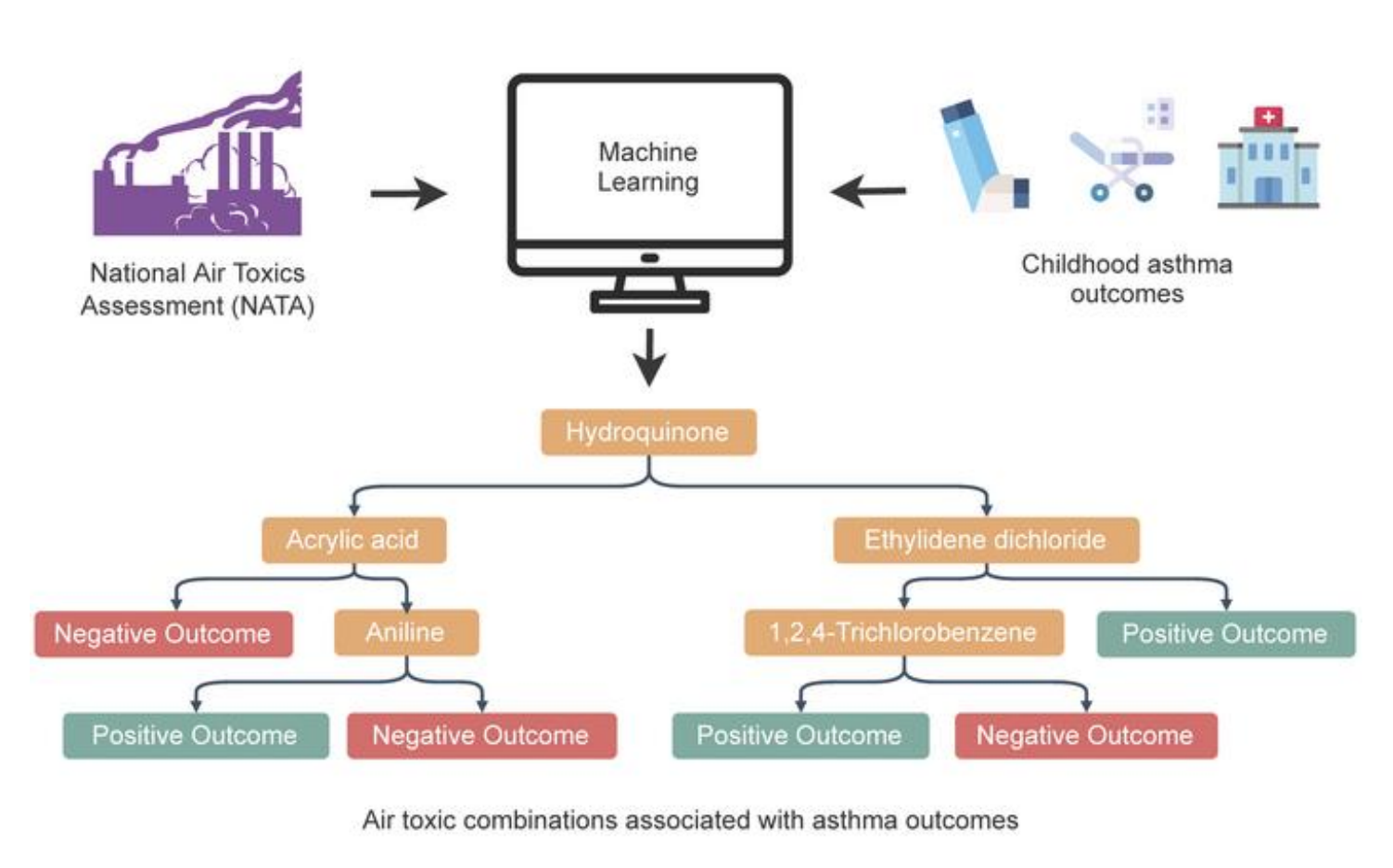
We identified combinations of early-life air pollutants associated with poor asthma outcomes in later childhood by applying a novel machine-learning method called Data-driven ExposurE Profile extraction (DEEP). Read more about it here in the Journal of Clinical Investigation.
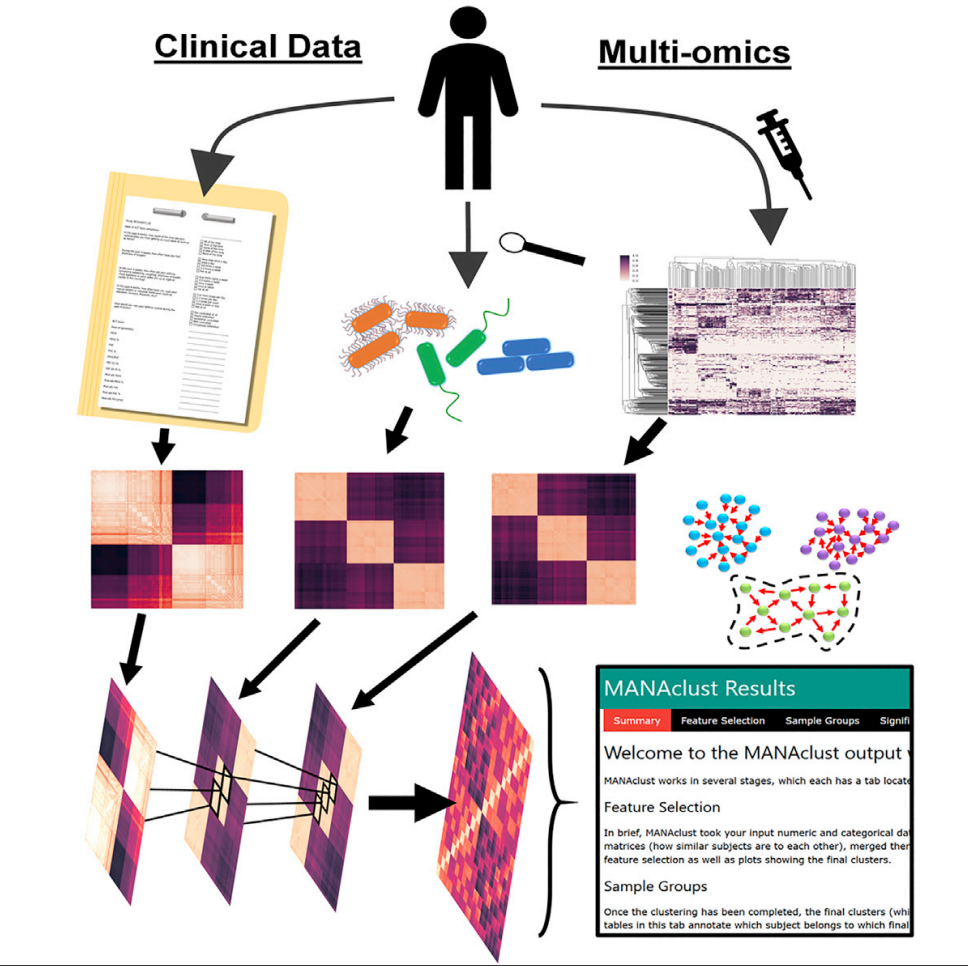
Merged Affinity Network Association Clustering (MANAclust) is a coding-free, automated pipeline developed by us that enables integration of multi-omic and clinical data clustering to identify disease endotypes. Full article in Cell Reports here.
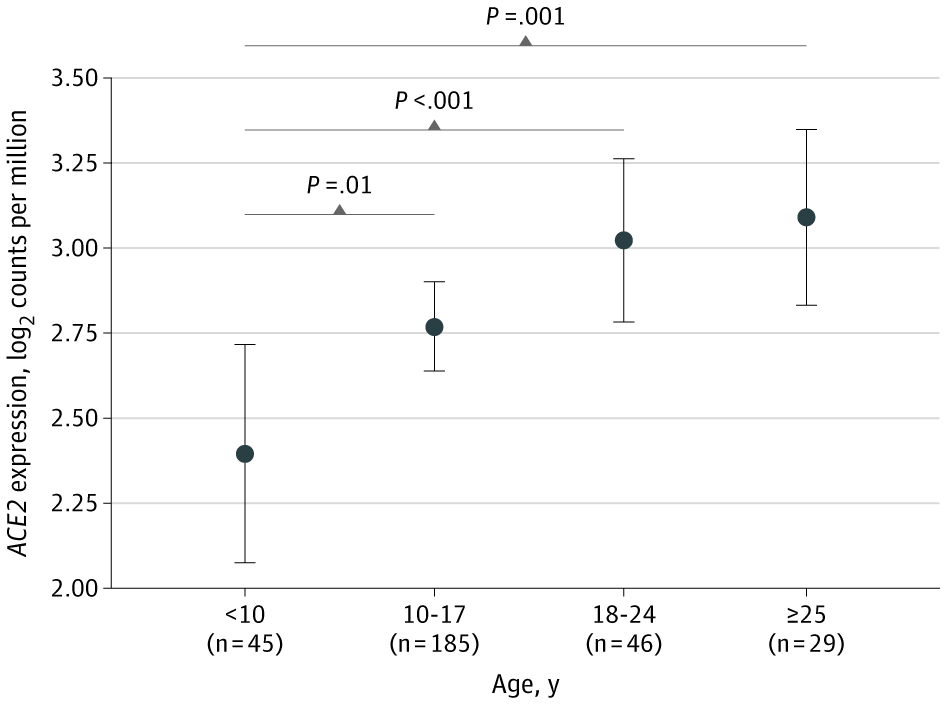
In this publication in JAMA, we report that nasal gene expression of ACE2, the receptor that the coronavirus SARS-CoV-2 uses to enter the host, is lowest in young children and increases with age. This may partially explain why children have suffered less from COVID-19. Clear here for the full text.

We systematically examined the transcriptome and microbiome of the upper and lower airway in children with severe asthma and controls to identify airway microbiota associated with host inflammatory gene expression in severe asthma. Click here for the full text.
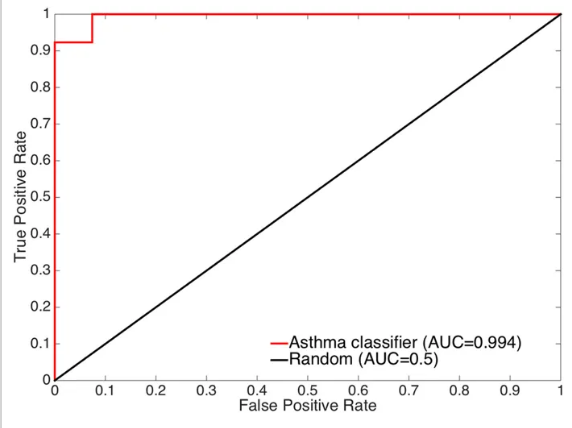
Using machine learning and nasal RNA sequence data from our well-characterized asthma cohorts, we identified and tested a nasal brush-based classifier of asthma. Click here to read the article.
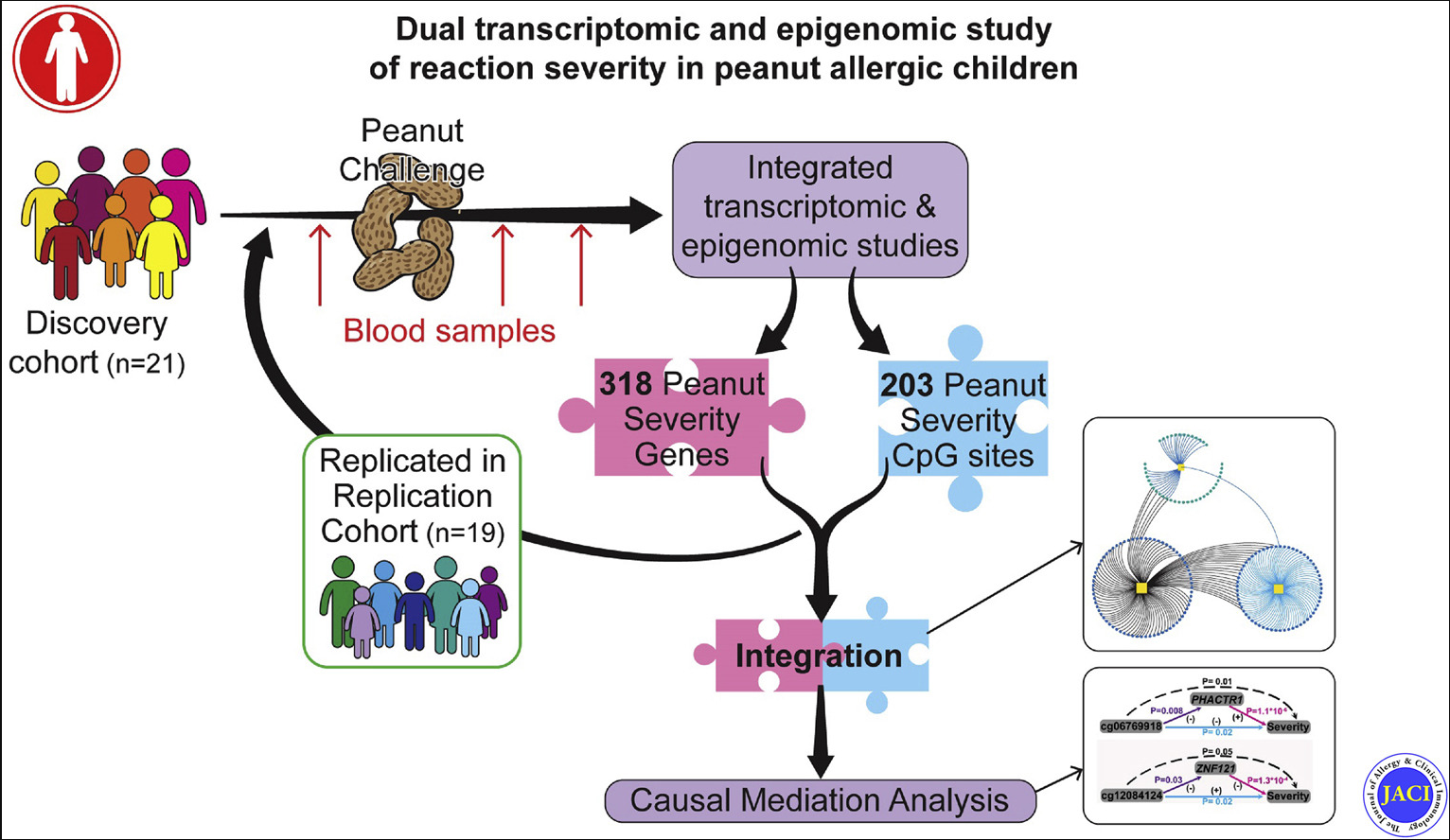
Our parallel transcriptomic and epigenomic study of peripheral blood from peanut allergic children undergoing peanut challenge identified genes, CpG loci, and causal interactions between them linked to allergic reaction severity. Read more about it here.
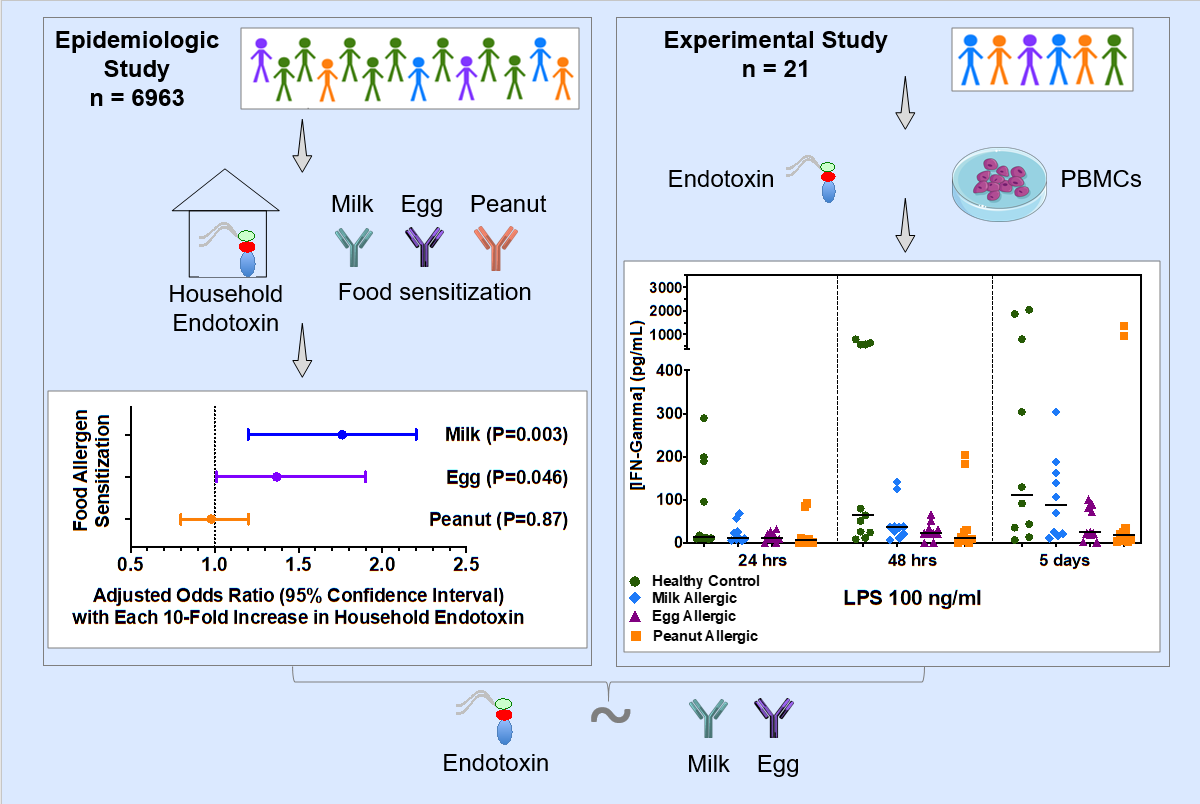
This complementary epidemiological and experimental study revealed that higher household endotoxin is associated with increased odds of milk and egg sensitization, and there is higher altered cytokine responsiveness to endotoxin in PBMCs from individuals with milk and egg allergy. Read more here.
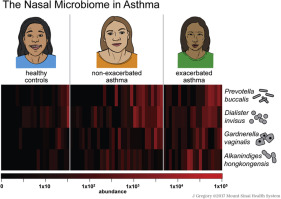
Our research group has characterized how the nasal microbiome varies with asthma activity. Read more here.
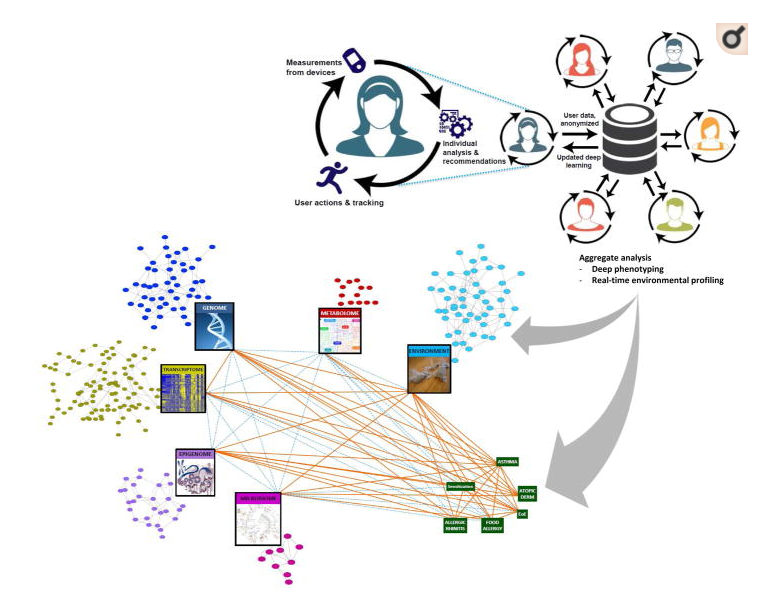
We examined applications of system-wide profiling to asthma and allergy and provided perspective on building network models to integrate multiscale data, including data from individually captured personal health profiles. Read more here.
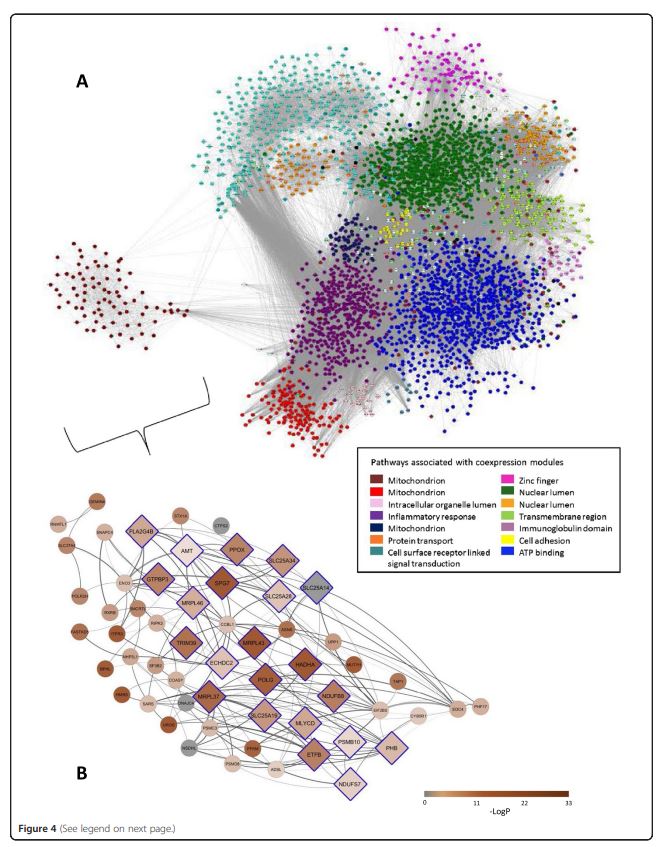
Through integrated genome-wide association study and network analysis of hay fever in over 5000 North Americans, we identified genetic loci linked to mitochondrial pathways. Click here to read the article.
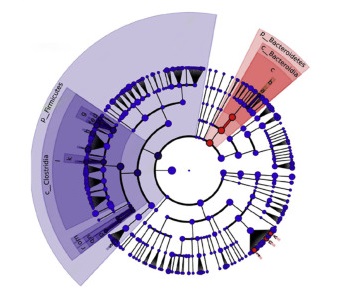
Our examination of early-life gut microbiota in milk allergic children from a longitudinal, multicenter observational study showed that Clostridia and Firmicutes are associated with resolution of milk allergy. Click here for more information.

We constructed causal networks using peripheral blood transcriptomes of peanut allergic children undergoing randomized, double-blind, placebo-controlled oral challenges to detect key drivers and putative therapeutic targets of peanut allergic reactions. Read more here.

We found that food-based vitamin D — but not supplemental vitamin D– in maternal diets during pregnancy was associated with lower rates of hay fever in children at school age. Read more here.
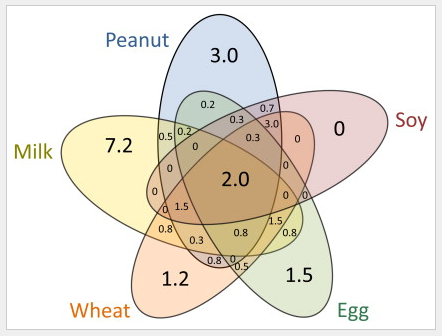
Our examination of maternal dietary habits during pregnancy in over 1000 mother-child pairs supports that pregnant women should not avoid specific foods during pregnancy to reduce the risk of their children developing asthma and allergies. Click here for the full text.
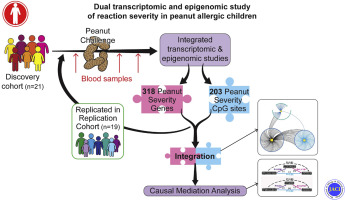
Our study of a large epidemiologic cohort revealed a greater mechanistic understanding of the genetics and epigenetics underlying the severity of peanut allergic reactions. Read More.